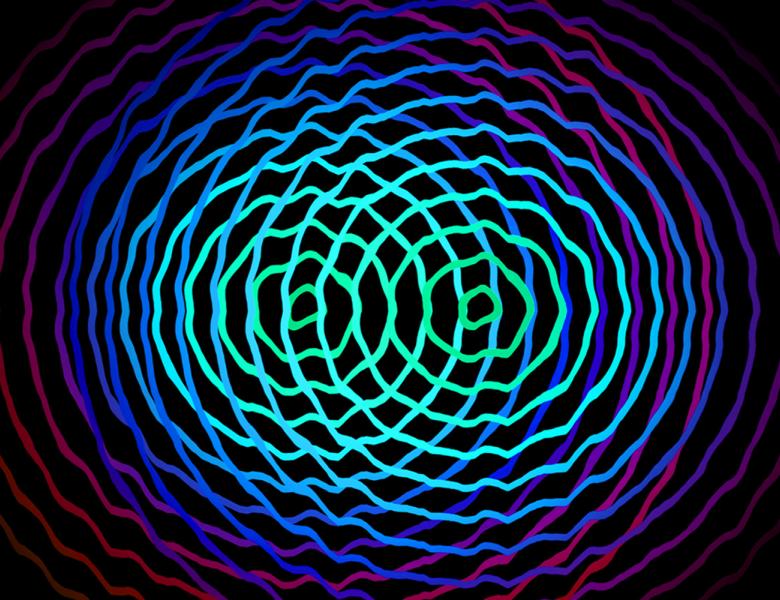
Abstract
Hamiltonian learning is the problem of learning a geometrically local N-qubit Hamiltonian H to precision ɛ, supposing we are given copies of its Gibbs state ρ = exp(-βH) / Tr(exp(-βH)) at a known inverse temperature β. I will present an algorithm that solves this problem with optimal sample complexity (number of copies of ρ needed) and optimal time complexity in the high-temperature (low β) regime. This improves on the previous best algorithm given by Anshu et al. (FOCS 2020), for the high-temperature regime. This work was done jointly with Jeongwan Haah and Robin Kothari.