Image
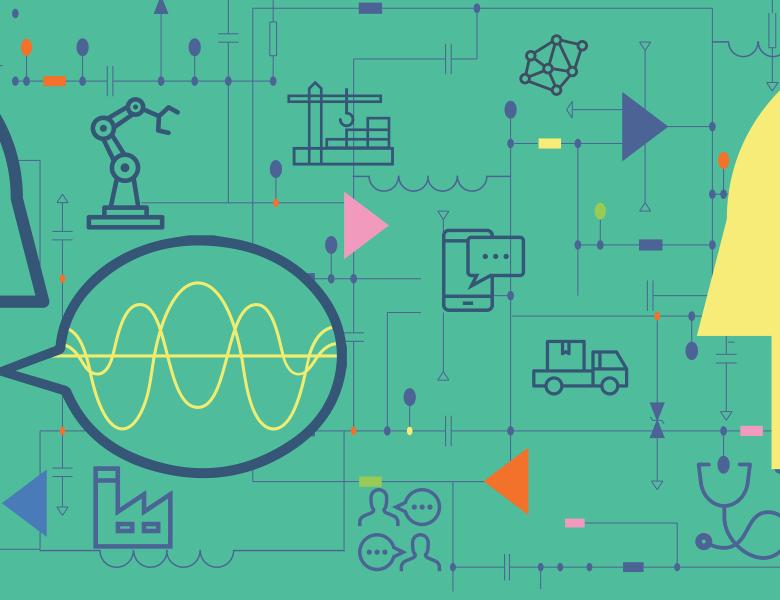
We introduce the problem of k-chasing of convex functions, a simultaneous generalization of both the famous k-server problem in R^d, and of the problem of chasing convex bodies and functions. Aside from fundamental interest in this general form, it has natural applications to online k-clustering problems with objectives such as k-median or k-means. We also introduce a parallel question of top-k action regret minimization in the realm of online convex optimization. In both settings, we design online algorithms and we prove lower bounds. We demonstrate that these problems exhibit a rich landscape of behavior.
This is joint work with Sebastien Bubeck and Mark Sellke.