Image
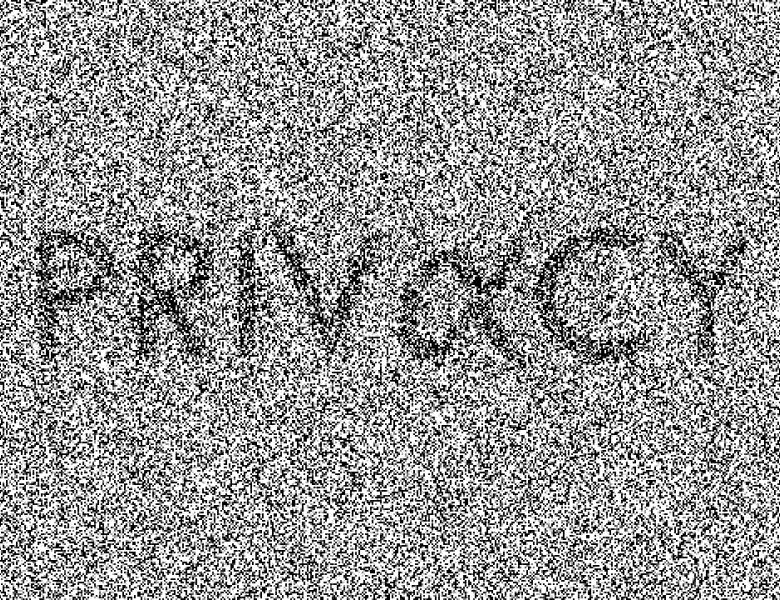
In this talk, I will present upper and lower bounds on the excess risk for differentially private stochastic convex optimization in non-Euclidean settings, in particular for $\ell_p$ spaces. Interestingly, our upper bounds for the ell_1 case are near dimension independent, and for any $1<p\leq 2$ we obtain a sharp transition of the excess risk, showing a polynomial dependence on dimension is necessary. Our algorithms are guaranteed to succeed both in expectation and with high probability. If time allows, I will discuss more recent work involving nonconvex losses and other related problems. Based on joint work with Raef Bassily, Mike Menart and Anupama Nandi.