Image
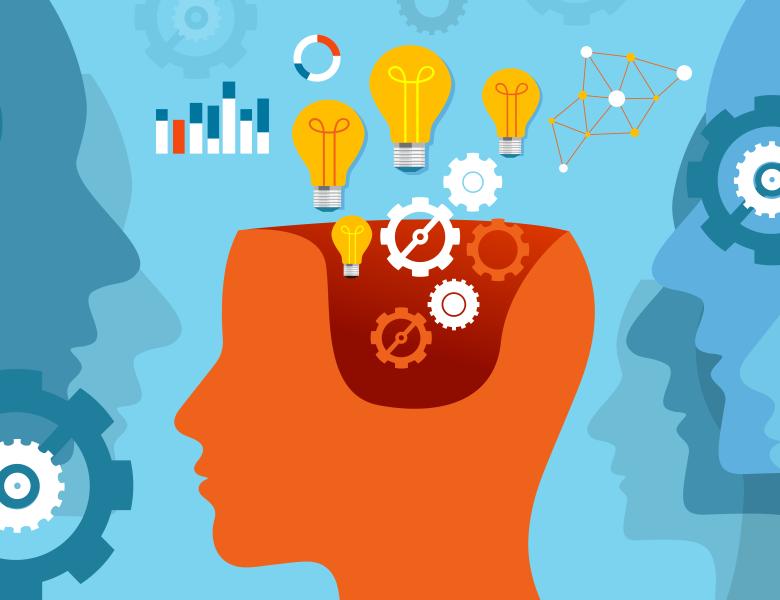
To solve complex tasks, especially those requiring multi-step or compositional reasoning and computation, autoregressive generation produces a Chain-of-Thought that ultimately leads to the desired answer. In this talk, I will discuss a formal framework for studying this emerging learning paradigm, both when the chain-of-thought is observed and when training only on prompt-answer pairs, with the chain-of-thought latent. We shall see how attention naturally arises as a key ingredient for "universal'' autoregressive learning with Chain-of-Thought. Central to our development is that iterating a fixed (time-invariant) next-token generator allows for sample complexity independent of the Chain-of-Thought length.