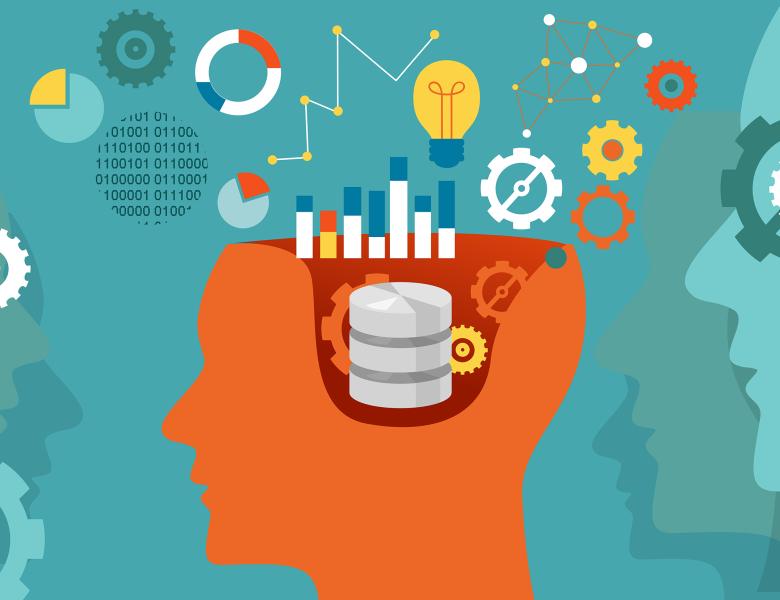
Abstract
Truthfulness is an important property of calibration measures, ensuring that the forecaster is not incentivized to exploit the system with deliberately poor forecasts. This makes it an essential desideratum for calibration measures, alongside typical requirements, such as soundness and completeness. It is surprising then that truthfulness of calibration measures has not been a subject of systematic study to date. In this talk, we introduce the concept of truthful calibration measures and conduct a taxonomy of existing calibration measures and their truthfulness. Perhaps surprisingly, we find that all of them are far from being truthful. That is, under existing calibration measures, there are simple distributions on which a polylogarithmic (or even zero) penalty is achievable, while truthful prediction leads to a polynomial penalty. We then introduce a new calibration measure termed the Subsampled Smooth Calibration Error (SSCE) under which truthful prediction is optimal up to a constant multiplicative factor.