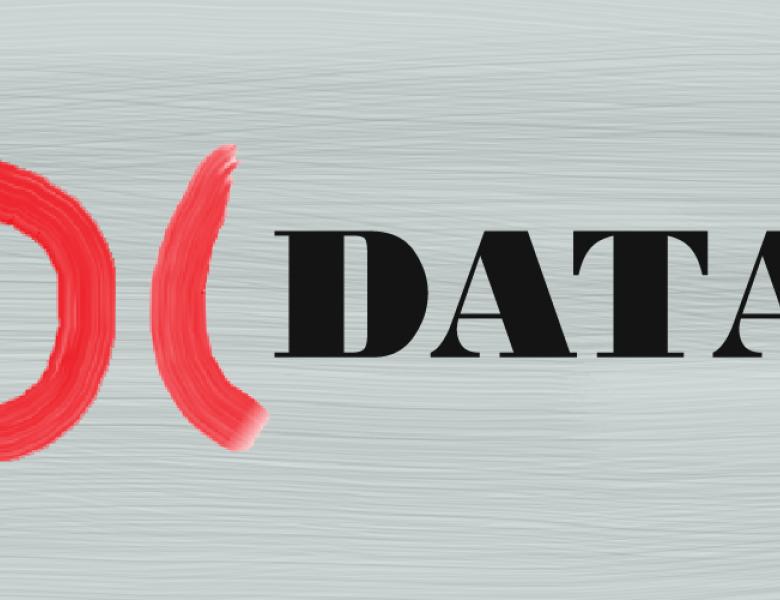
Abstract
We study large-scale regression analysis where both the number of variables, p, and the number of observations, n, may be large and in the order of millions or more. This is very different from the now well-studied high-dimensional regression context of "large p, small n". For example, in our "large p, large n" setting, an ordinary least squares estimator may be inappropriate for computational, rather than statistical, reasons. In general one must seek a compromise between statistical and computational efficiency. Furthermore, in contrast to the common assumption of signal sparsity for high dimensional data, here it is the design matrices which are typically sparse in applications. Our approach for dealing with this large, sparse data is based on b-bit min-wise hashing (Li and Koenig, 2011). This can be viewed as a dimensionality reduction technique for a sparse binary design matrix; our variant, which we call min-wise hash random-sign mapping (MRS mapping) also handles the real-valued case, and is more amenable to statistical analysis. For both linear and the logistic models, we give finite-sample bounds on the prediction error of procedures which perform regression in the new lower-dimensional space after applying MRS mapping. In particular, we show that the average prediction error goes to 0 asymptotically as long as q*l(b)^2/n goes to 0 for n to infinity, where q is the maximal number of non-zero entries in each row of the design matrix and l(b) is the l2-norm of an optimal coefficient for the linear predictor. We also show that ordinary least squares or ridge regression applied to the reduced data can actually allow us to capture interactions in the original data. In addition to the results on prediction, we show how one can recover measures of the importance of the original variables. A simulation study and some applications help illustrate the circumstances under which we can expect MRS mapping to perform well.