Image
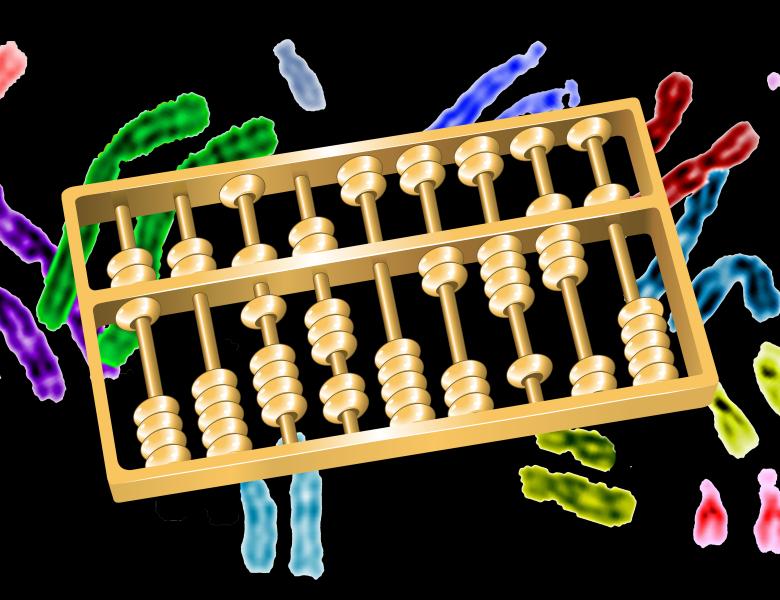
Gaussian processes provide a convenient and flexible class of non-parametric model for temporal and spatial data. We are applying Gaussian processes in a range of biological applications involving high-throughput time course data, e.g. modeling the elongation dynamics of polymerase, uncovering mRNA production delays, inferring regulatory networks and most recently identifying perturbations and bifurcations from high-throughput expression data. I will provide an overview of Gaussian process inference and describe some of our recent work in modeling gene expression dynamics.