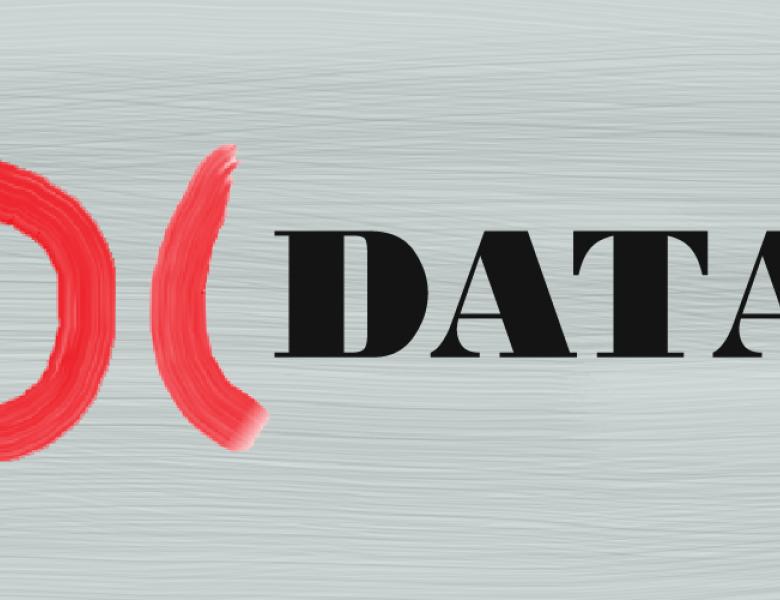
Abstract
Varying coefficient models have been successfully applied in a number of scientific areas ranging from economics and finance to biological and medical science. Varying coefficient models allow for flexible, yet interpretable, modeling when traditional parametric models are too rigid to explain heterogeneity of sub-populations collected. Currently, as a result of technological advances, scientists are collecting large amounts of high-dimensional data from complex systems which require new analysis techniques. We focus on the high-dimensional linear varying-coefficient model and develop a novel procedure for estimating the coefficient functions in the model based on penalized local linear smoothing. Our procedure works for regimes which allow the number of explanatory variables to be much larger than the sample size, under arbitrary heteroscedasticity in residuals, and is robust to model misspecification as long as the model can be approximated by a sparse model. We further derive an asymptotic distribution for the normalized maximum deviation of the estimated coefficient function from the true coefficient function. This result can be used to test hypotheses about a particular coefficient function of interest, for example, whether the coefficient function is constant, as well as construct confidence bands for covering the true coefficient function. Construction of the uniform confidence bands relies on a double selection technique that guards against omitted variable bias arising from potential model selection mistakes. We demonstrate how these results can be used to make inference in high-dimensional dynamic graphical models. Joint work with Damian Kozbur.