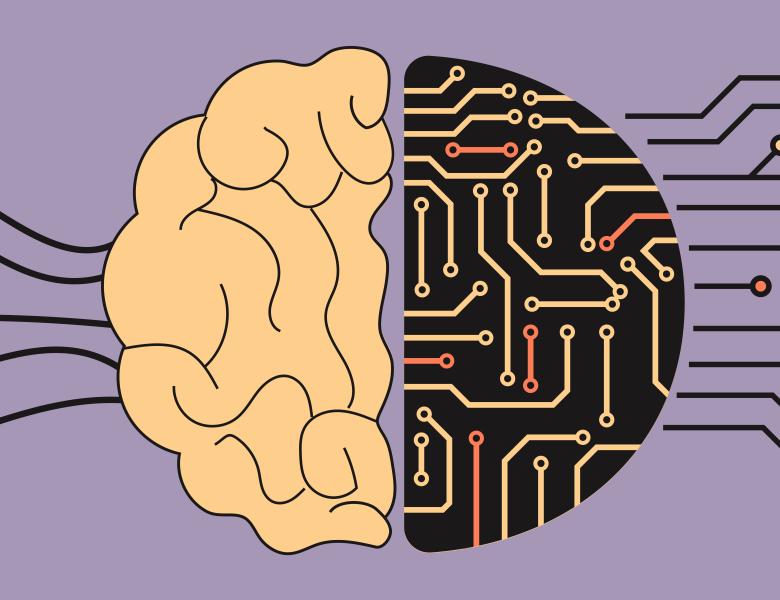
Abstract
We argue that a well-trained reinforcement learning (RL) agent planning over a long time-horizon would likely commandeer all human infrastructure. We argue that for a long-term RL agent, the expected value of its reward signal would likely be near-maximal if it sought to commandeer all human infrastructure, whereas the expected value of its reward signal would be bounded above if it did not do so. Next, we argue that a well-trained RL agent would likely aim to maximize the expectation of its reward signal: if it did not start with that motivation to begin with, its motivations would eventually be refined to that after it pursued value-of-information-motivated exploration. Next, we discuss how and why variants of RL agents might behave differently, and we outline potential safety cases for each variant: KL-constrained RL, myopic and contained RL, and pessimistic RL.