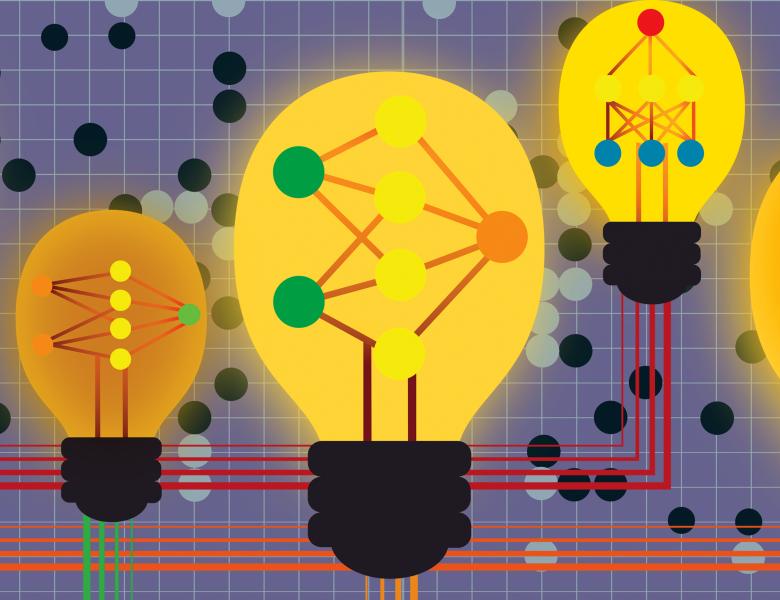
Abstract
In a variety of contexts such as peer review, peer grading, and crowd-sourcing we would like to reward agents for producing high quality information. Unfortunately, computing rewards by comparing to ground truth or gold standard is often cumbersome, costly, or impossible. Instead we would like to compare agent reports. A key challenge is that agents may strategically withhold effort or information when they believe their payoff will be based upon comparison with other agents whose reports will likely omit this information due to lack of effort or expertise. This talk will show how to translate machine learning solutions, which provide a forecast for an agent's report (given the other agents' reports), into provably incentive compatible mechanisms. The key theoretical technique is a variational interpretation of mutual information, which permits machine learning to estimate mutual information using only a few samples (and likely has other applications in machine learning even beyond strategic settings). Time-permitting, we will show empirically how augmenting information elicitation mechanisms with basic machine learning techniques can improve the ex post fairness of rewards, an important criteria for many applications. (No information theory knowledge will be assumed.)