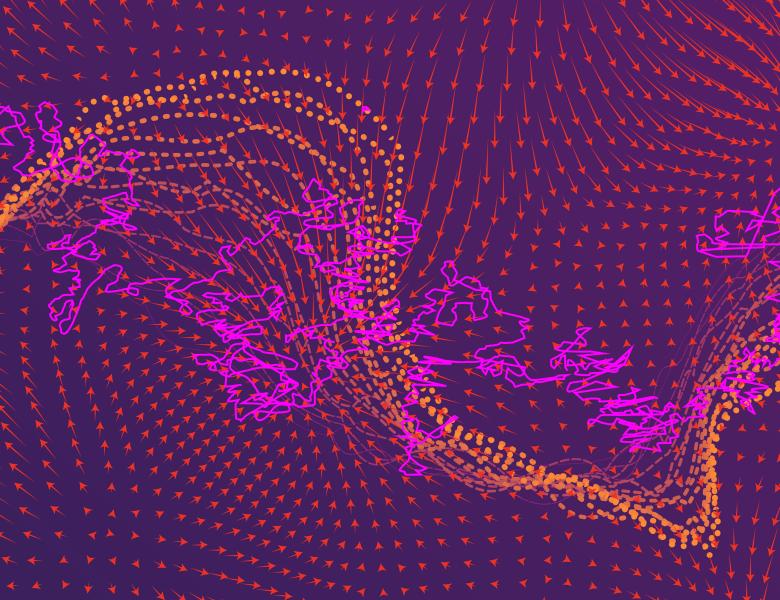
Abstract
We consider the problem of universal approximation of functions by two-layer neural nets with random weights that are ``nearly Gaussian'' in the sense of Kullback-Leibler divergence. This problem is motivated by recent works on lazy training, where the updates of stochastic gradient descent do not move the weights substantially from their i.i.d. Gaussian initialization. Our setting is the mean-field limit, where the finite population of neurons in the hidden layer is replaced by a continuous ensemble. We show that the problem can be phrased as global minimization of a free energy functional on the space of (finite-length) paths over probability measures on the weights. This functional trades off the $L^2$ approximation risk of the terminal measure against the KL divergence of the path with respect to an isotropic Brownian motion prior. We characterize the unique global minimizer and examine the dynamics in the space of probability measures over weights that can achieve it. In particular, we show that the optimal path-space measure corresponds to the F\"ollmer drift, the solution to a McKean-Vlasov optimal control problem closely related to the classic Schr\"odinger bridge problem. While the F\"ollmer drift cannot in general be obtained in closed form, thus limiting its potential algorithmic utility, we illustrate the viability of the analogous Langevin diffusion as a finite-time approximation under various conditions on entropic regularization. Specifically, we show that it closely tracks the F\"ollmer drift when the regularization is such that the minimizing density is log-concave, corroborating the laziness of training using SGD. We also derive log-Sobolev inequalities to characterize its suboptimality in the semi-concave and general cases.