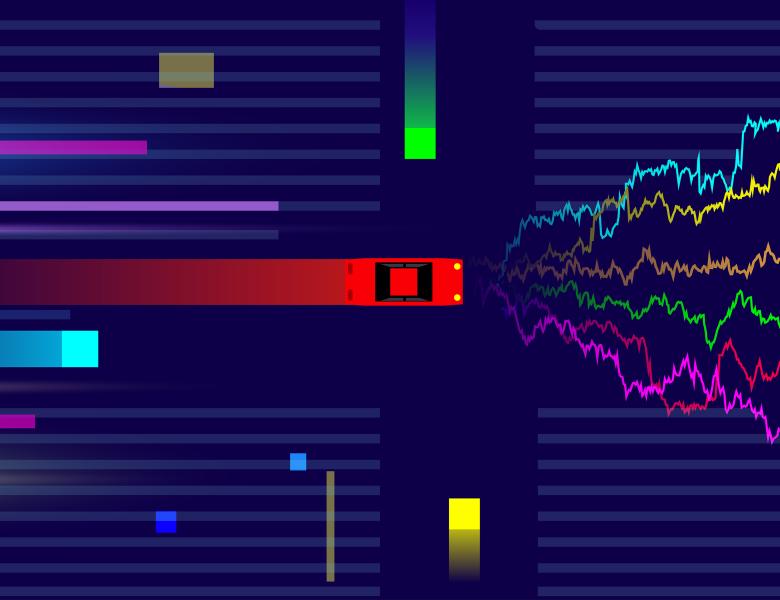
Abstract
Standard Markov decision process (MDP) features a single planner who observes the underlying state of a world and then acts. This talk will study a natural variant of this fundamental model, in which one agent observes the state whereas another agent acts. Such sequential interactions among different agents arise in various recommender systems such as ride-sharing platforms or content recommendations. When agents have different incentives, the state-informed agent can partially reveal information about the realized state to influence the actor at each round in order to steer their collective actions towards a desirable outcome, a problem which we coin Markov Persuasion Process (MPP) inspired by the celebrated recent literature of Bayesian persuasion. We will talk about both computational and reinforcement learning questions in MPP.