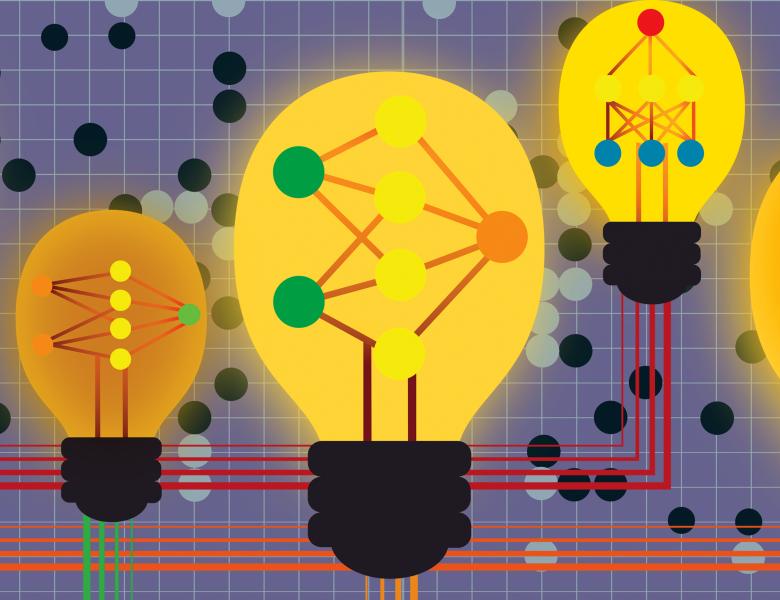
Abstract
(This is only a tentative abstract, subject to change)
We propose a multi-agent reinforcement learning dynamics, and analyze its convergence properties in infinite-horizon discounted Markov potential games. We focus on the independent and decentralized setting, where players can only observe the realized state and their own reward in every stage. Players do not have knowledge of the game model, and cannot coordinate with each other. In each stage of our learning dynamics, players update their estimate of a perturbed Q-function that evaluates their total contingent payoff based on the realized one-stage reward in an asynchronous manner. Then, players independently update their policies by incorporating a smoothed optimal one-stage deviation strategy based on the estimated Q-function. A key feature of the learning dynamics is that the Q-function estimates are updated at a faster timescale than the policies. We prove that the policies induced by our learning dynamics converge to a stationary Nash equilibrium in Markov potential games with probability 1. {Our results demonstrate that agents can reach a stationary Nash equilibrium in Markov potential games through simple learning dynamics under minimal information environment.