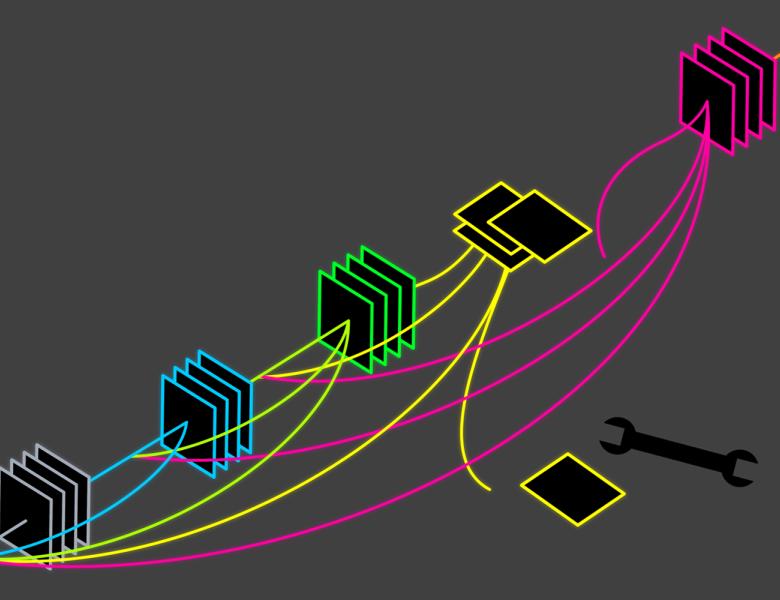
Abstract
With the advent of higher throughput and more accurate technologies to measure protein properties of interest, such as target binding to a drug, the time for machine learning to act synergistically with protein design is here. The obvious first place to do so is to replace the lab measurements with, for example, a deep neural network based predictive model. Then, one can ask how to invert that model to find desired protein sequences. Naively, inverting this model could be viewed as combinatorial optimization. However, one must take into account possibly heteroscedasctic uncertainty of the predictive model. Calibrating these uncertainties, even in region of the training data, has been tackled, but could be improved. Moreover, "further away" from the training data, the uncertainties are arbitrarily bad. How can we tackle the general design problem when the functions we are optimizing cannot even be trusted?