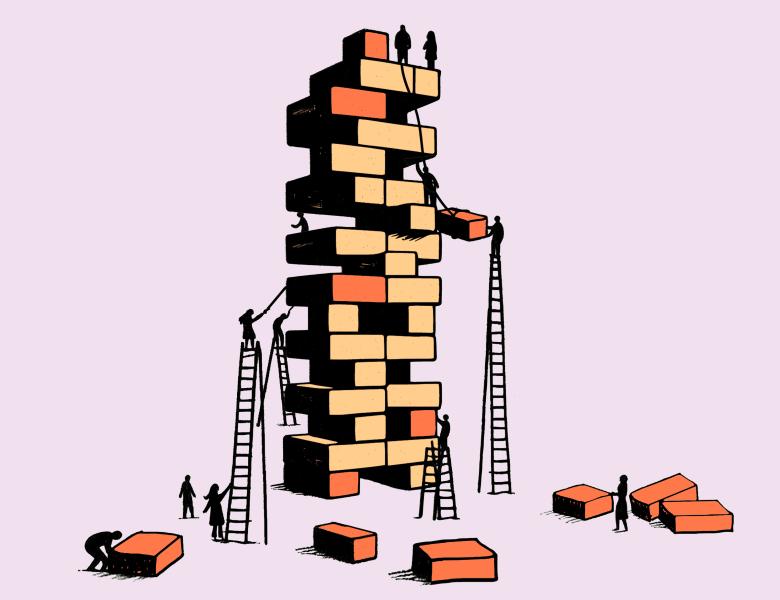
Abstract
Differential privacy has been established as a rigorous standard for protecting the privacy of individuals' data used in machine learning and statistical analyses. However, there exists a tension between differential privacy and accuracy, which particularly manifests in high-dimensional tasks, in the form of the so-called "curse of dimensionality". For the first part of the talk, we will discuss recent work achieving instance-adaptive error bounds which allow us to learn accurately with a dimension-free number of samples. For the second part of the talk, we will discuss fruitful connections between differential privacy and other forms of algorithmic stability, which played a role in the above results and beyond.