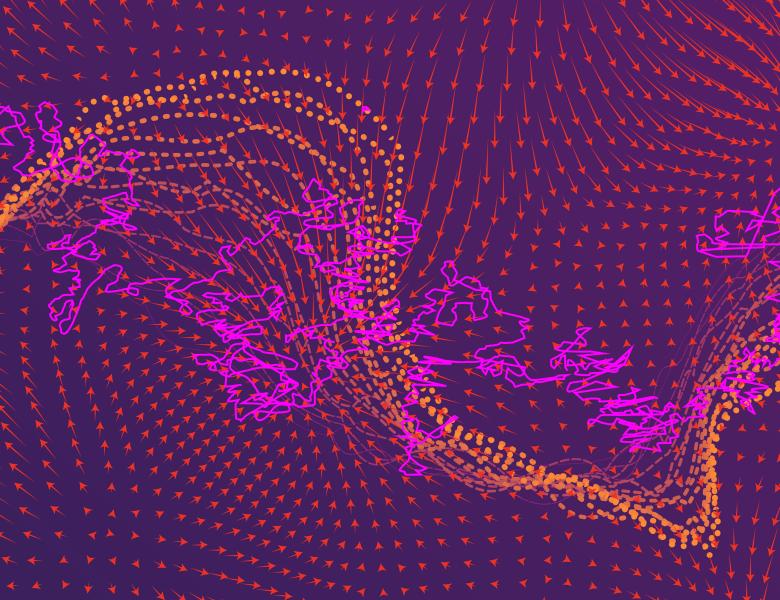
Abstract
We give lower bounds on the performance of two of the most popular sampling methods in practice, the Metropolis-adjusted Langevin algorithm (MALA) and multi-step Hamiltonian Monte Carlo (HMC) with a leapfrog integrator, when applied to well-conditioned distributions. Our main result is a nearly-tight lower bound of Ω˜(κd) on the mixing time of MALA from an exponentially warm start, matching a line of algorithmic results up to logarithmic factors and answering an open question of Chewi et. al. We also show that a polynomial dependence on dimension is necessary for the relaxation time of HMC under any number of leapfrog steps, and bound the gains achievable by changing the step count. Our HMC analysis draws upon a novel connection between leapfrog integration and Chebyshev polynomials, which may be of independent interest. Joint work with Yin Tat Lee and Ruoqi Shen