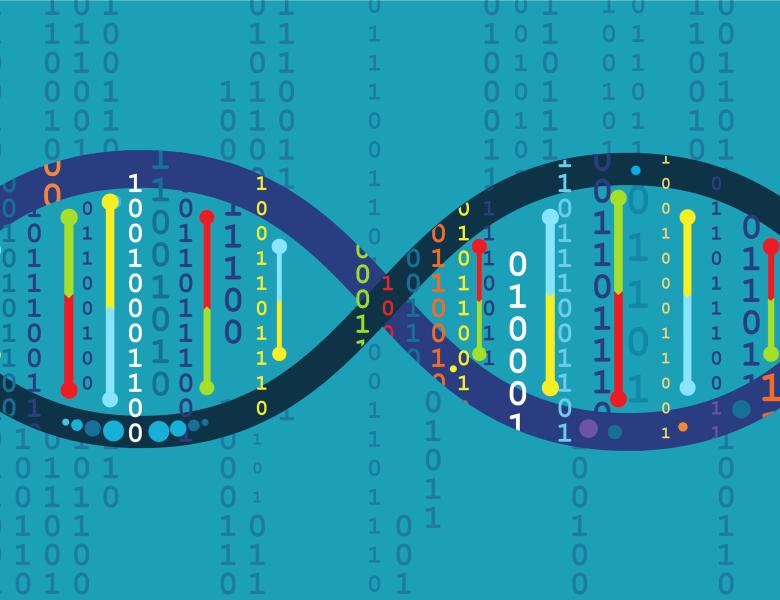
Abstract
Multi-step disease and prescription trajectories are key to the understanding of human disease progression patterns and their underlying molecular level etiologies. The number of human protein coding genes is small, and many genes are presumably impacting more than one disease, a fact that complicates the process of identifying actionable variation for use in precision medicine efforts. We present approaches to the identification of frequent disease and prescription trajectories from population-wide healthcare data comprising millions of patients and corresponding strategies for linking disease co-occurrences to genomic individuality. In the work we carry out temporal analysis of clinical data in a life-course oriented fashion. We use data covering 7-10 million patients from Denmark collected over a 20-40 year period and use them to “condense” millions of individual trajectories into a smaller set of recurrent ones. Such sets represent patient subgroups sharing longitudinal phenotypes that could form a basis for differential treatment designs of relevance to individual patients. Individual disease and prescription trajectories can also be used as input to machine learning approaches for risk and time to event prediction.