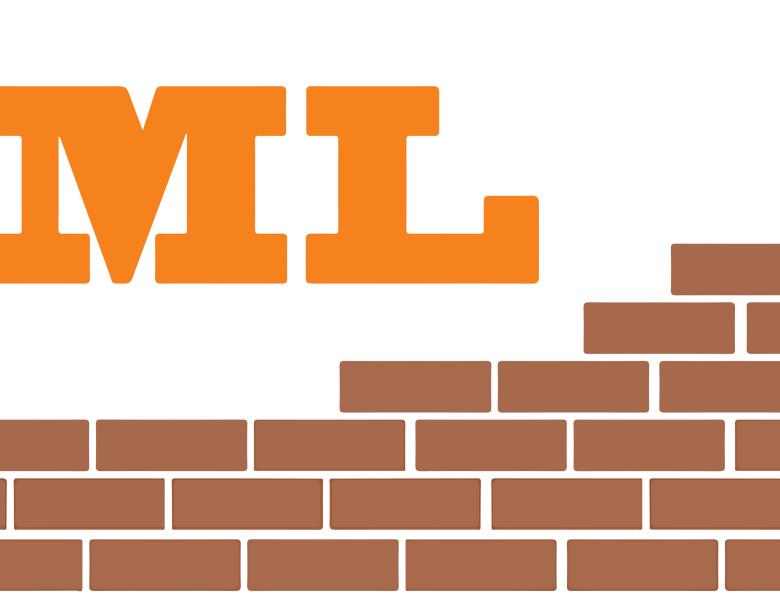
Abstract
Policy evaluation is a crucial step in many reinforcement-learning problems, which estimates a value function that predicts long-term value of states for a given policy. In this talk, we present stochastic variance reduction algorithms that learn value functions from a fixed dataset, which is shown to have (i) guaranteed linear convergence rate, and (ii) linear complexity (in both sample size and feature dimension), under the condition of linear function approximation and possibly off-policy learning as well as eligibility traces. In particular, we transform the policy evaluation problem into an empirical (quadratic) saddle-point problem and apply stochastic variance reduction methods in the primal-dual space. Interestingly, the algorithms converge linearly even when the quadratic saddle-point problem has only strong concavity but no strong convexity. Numerical experiments on random MDPs and on Mountain Car demonstrate improved performance of our algorithms.