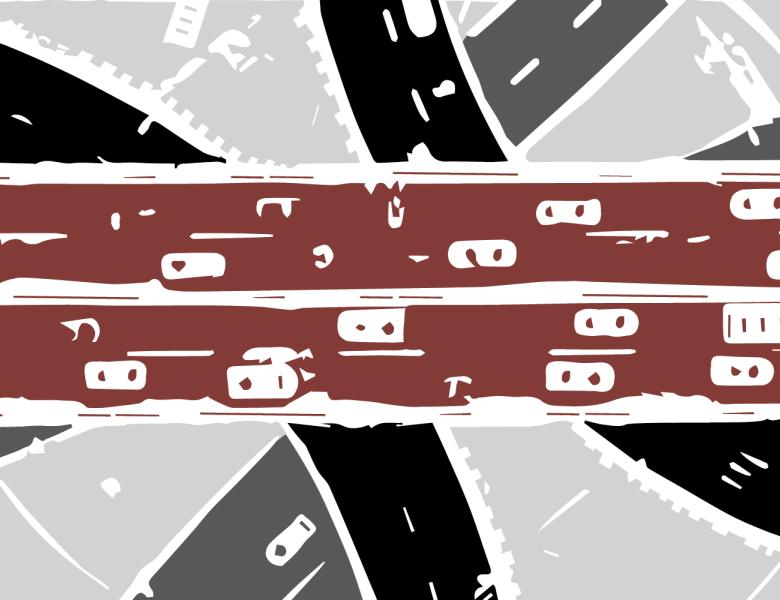
Abstract
As the penetration of renewable increases and conventional generators retire, the reliability concerns on balancing real-time demand and supply motivate the utilization of demand-side resources. However, residential demands which consist the most significant share of electricity demand are still underutilized in grid operation. Although both industry and academia are conducting pilot studies and developing theories respectively, they have not been serendipitously connected. This work performs a case study of an actual pilot study and its dataset. Specifically, we train learning models to model users’ behavior to enhance DR performance for peak load reduction, and we also develop a combinational multi-armed bandit (MAB) algorithm to exploit demands while exploring stochastic models of these demands. The MAB problem is different from the classic ones in the sense that the objective function is non-monotone since the goal is to maximize reliability, that is, to minimize the difference between the total load reduction and a target value. Thus we propose a learning algorithm and prove that the proposed algorithm achieves O(log T) regret given a static target, and o(T) regret when the target is time-varying. Our preliminary results show that the application of modern learning techniques can help improve the performance of DR pilots in practice. We hope that this work can bridge the gap between DR pilots and theoretical analysis, and eventually unlocking the potential residential demands in grid operation.
This work is joint with Yingying Li (Harvard University), Qinran Hu (Harvard University) Jun Shimada (ThinkEco Inc), Alison Su (ThinkEco Inc)