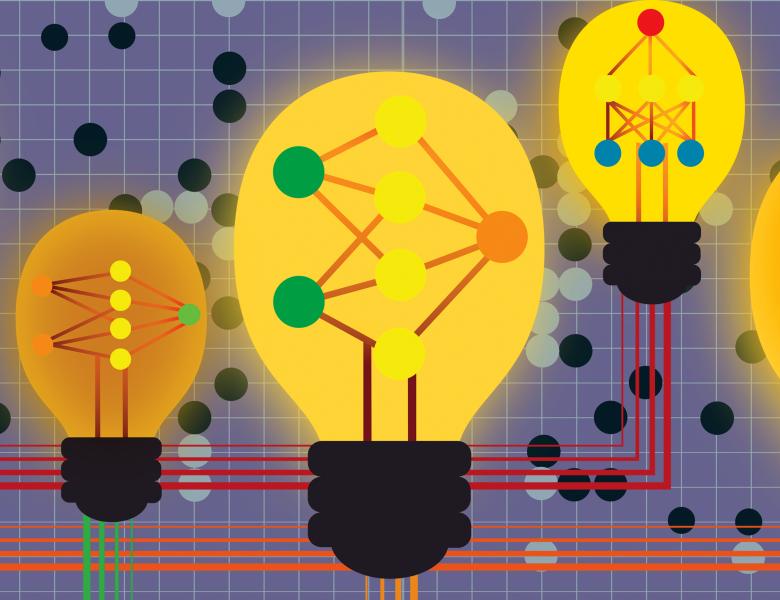
Abstract
In over the last two decades we have developed good understanding how to quantify the impact of strategic user behavior on overall performance in many games (including traffic routing as well as online auctions). Early work focused on evaluating the quality of Nash equilibria. However, it turns out that the resulting bounds extend to learning out comes in repeated games under pretty general assumptions.
In this talk we will review these results, which mostly assume that learners satisfy versions of the no-regret property after a long enough play. We will also discuss pros and cons of no-regret as a behavioral assumption on learning outcomes, as well as limitations of assuming no-regret as the main condition players achieve as they are learning.