Image
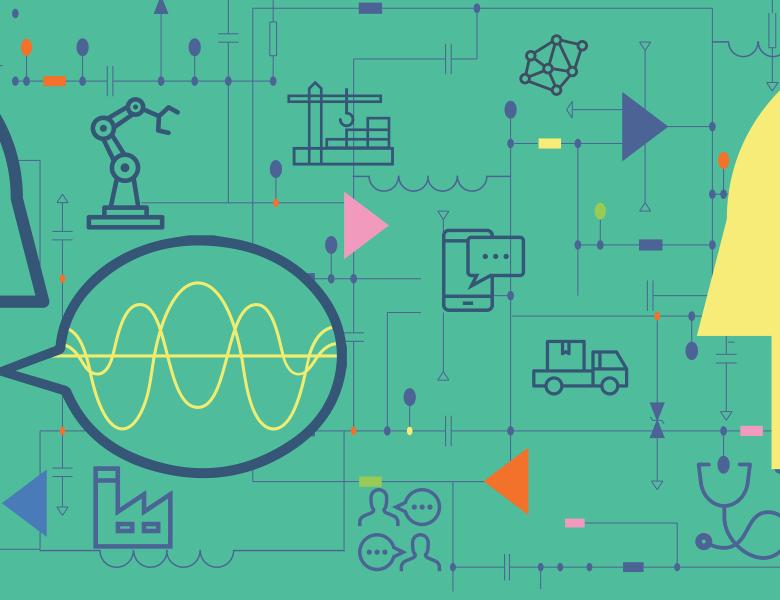
Offline batch RL has huge promise to enable reinforcement learning to be used more widely, in high stakes settings where exploration is costly, or in complex engineering settings where implementing online RL is a large change to existing practices. While there has been significant attention to accurately estimating the performance of a single decision policy, learning the best policy to deploy in the future remains an open challenge. I’ll discuss some of our recent work showing that pessimism can help ensure that model-free methods compute policies well supported within the available dataset, and allows us to obtain strong theoretical results under more general settings.