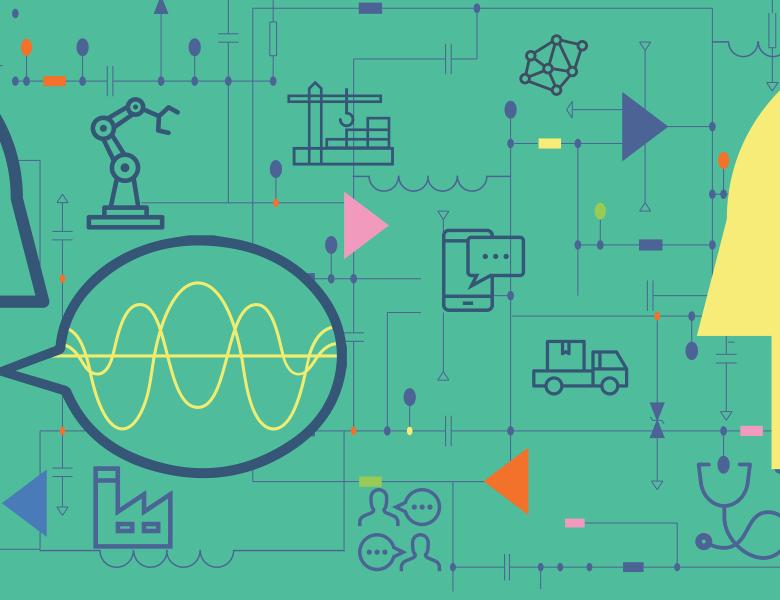
Abstract
While Reinforcement Learning has achieved impressive empirical performance in many single-agent (e.g., Go, StarCraft) and multi-agent games (e.g., DoTA), many challenges remain. For now, brute-force approaches with massive amounts of computational resources are often used to learn strong agents, which may not be necessary if the hidden structures are employed. In this talk, we introduce our recent works for policy training that attempt to reduce the high complexity in multi-agent collaborations by decomposing into their intrinsic structures. While we mainly test our methods in StarCraft Multi-agent Challenge and Contract Bridge Bidding, the structure utilized in the proposed algorithms is quite general and can be used in many other problems. In addition, we will also introduce a very effective criterion for efficient exploration in RL.