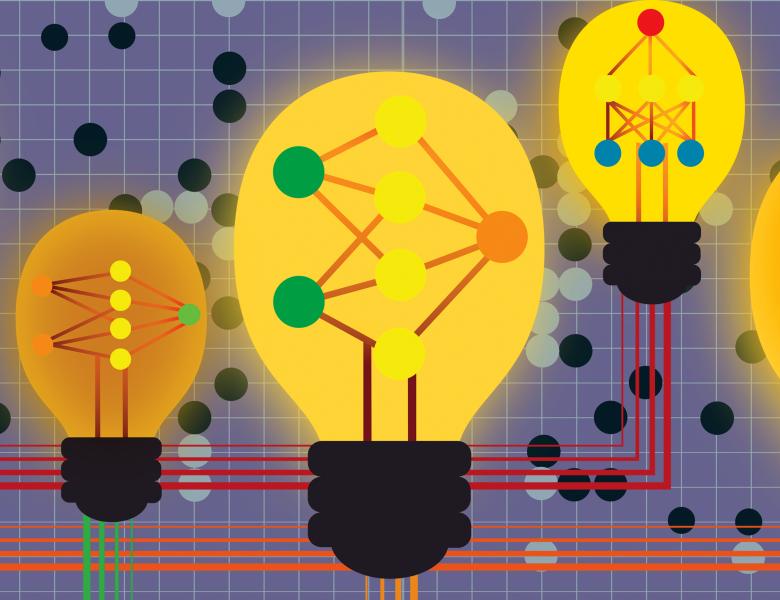
Abstract
Abstract: In this talk I will discuss a few lines of work involving learning, incentivizing improvement, and fairness in the presence of strategic behavior. In the first, we consider online linear classification: agents (who all wish to be classified as positive) arrive one at a time, observe the current decision rule, and then modify their observable features to get a positive classification if they can do so at a cost less than their value for being positively classified. A particular challenge here is that updates made by the learning algorithm will change how agents behave, and will do so in a non-convex and discontinuous manner. In the second line we consider the simpler offline (batch) setting, but with the twist that agents have both gaming and improvement actions, and the decision-maker would like to incentivize agents to become positive (e.g., to create more qualified loan applicants). In the third line, we consider a pure improvement setting, and examine several fairness objectives. This is joint work with Saba Ahmadi, Hedyeh Beyhaghi, and Keziah Naggita.