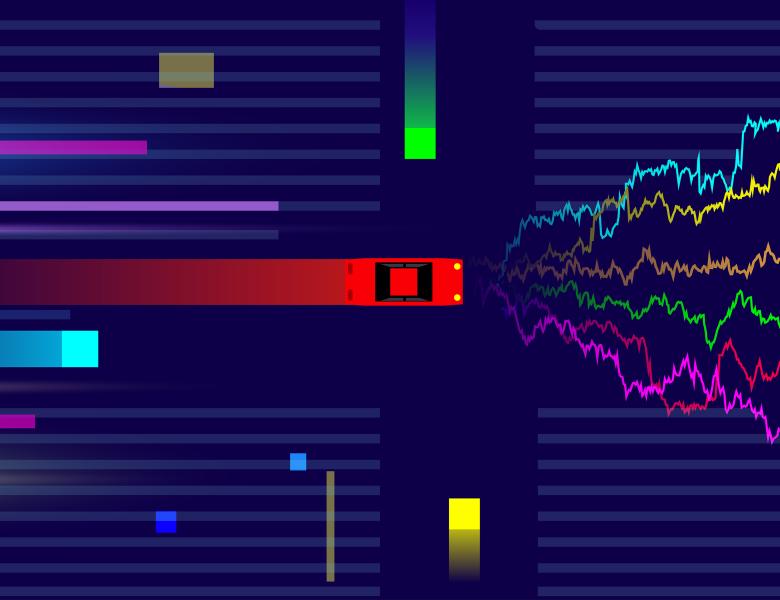
Abstract
Making use of modern black-box AI tools such as deep reinforcement learning is potentially transformational for safety-critical systems such as data centers, the electricity grid, transportation, and beyond. However, such machine-learned algorithms typically do not have formal guarantees on their worst-case performance, stability, or safety and are typically difficult to make use of in distributed, networked settings. So, while their performance may improve upon traditional approaches in “typical” cases, they may perform arbitrarily worse in scenarios where the training examples are not representative due to, e.g., distribution shift, or in situations where global information is unavailable to local controllers. These represent significant drawbacks when considering the use of AI tools in safety-critical networked systems. Thus, a challenging open question emerges: Is it possible to provide guarantees that allow black-box AI tools to be used in safety-critical applications? In this talk, I will provide an overview of a variety of projects from my group that seek to develop robust and localizable tools combining model-free and model-based approaches to yield AI tools with formal guarantees on performance, stability, safety, and sample complexity.