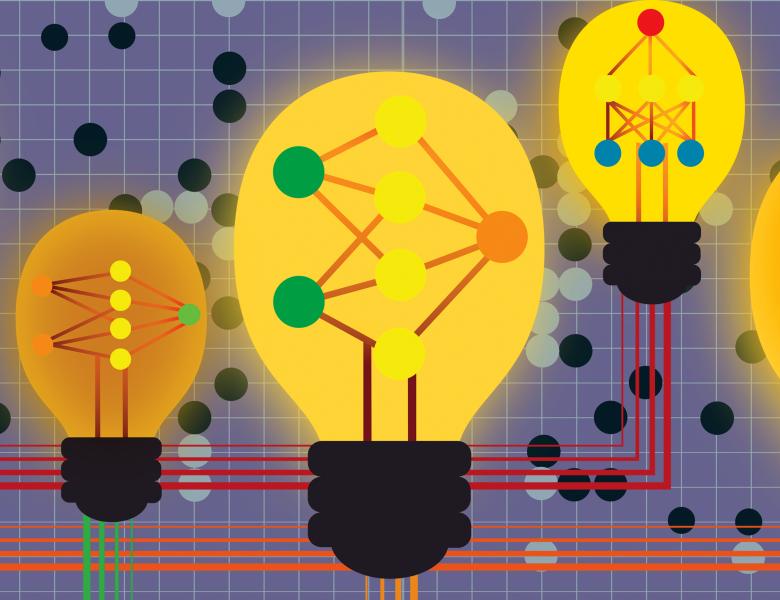
Abstract
The extragradient method by Kopelevich (1976) is one of the most popular methods for solving smooth, convex-concave saddle point problems. Despite its long history and intensive attention from the optimization and machine learning community, the following major problem remains open. What is the last-iterate convergence rate of the extragradient method for smooth, convex-concave saddle point problems with constraints? We resolve this open problem by showing a tight O(1/\sqrt{T}) last-iterate convergence rate for arbitrary convex constrained sets. Our result also holds for the more general monotone variational inequality problems. Our analysis combines the power of the sum-of-squares programming with the low dimensionality of the update rule of the extragradient method. Our approach may be useful in analyzing other iterative methods. Based on joint work with Argyris Oikonomou and Weiqiang Zheng.