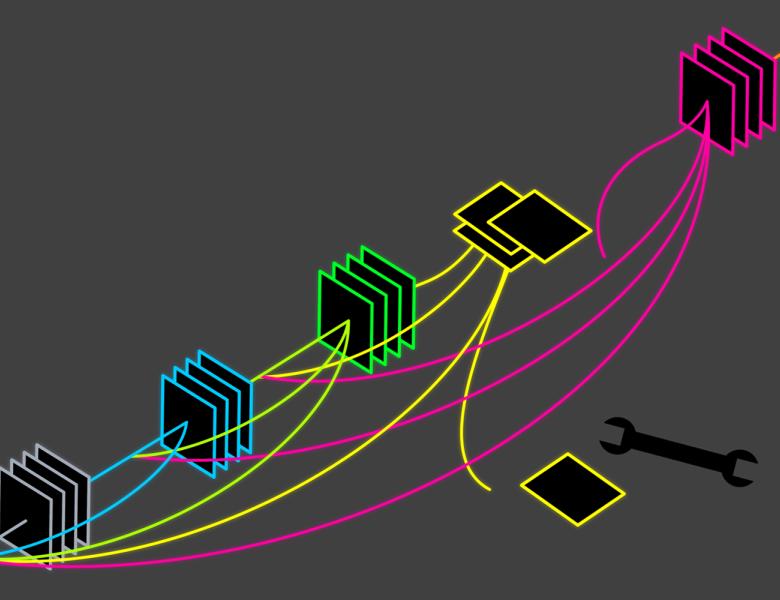
Abstract
Recent investigations of infinitely-wide deep neural networks have given rise to connections between deep nets, kernels, and Gaussian processes. Still, there is a gap to understanding the dynamics of finite-width neural networks in common optimization settings. I discuss how the choice of learning rate is a crucial factor to be considered and naturally classifies gradient descent dynamics of deep nets into two classes (a lazy regime and a catapult regime) which are separated by a sharp (phase) transition as networks become wider. I discuss the distinct phenomenological signatures of the two phases and how they are elucidated in a class of solvable simple models we analyze.