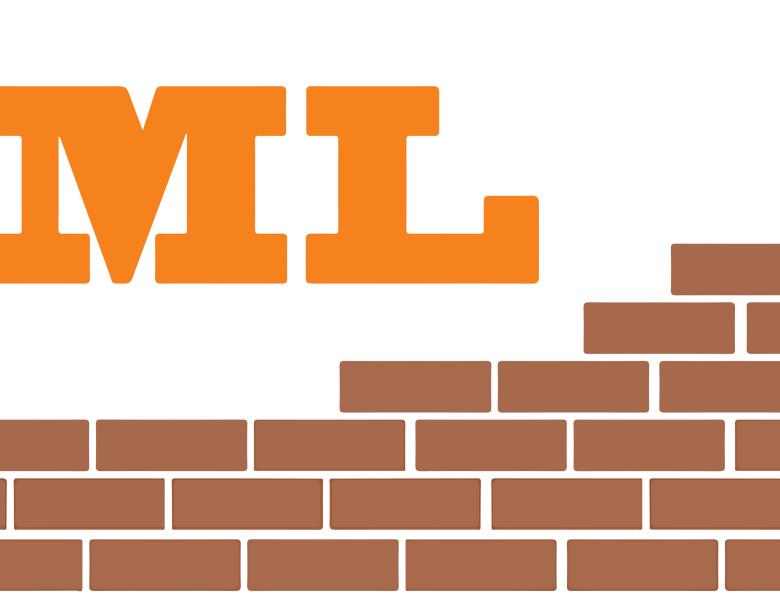
Abstract
The status quo in visual recognition is to learn from batches of unrelated Web photos labeled by human annotators. Yet cognitive science tells us that perception develops in the context of acting and moving in the world---and without intensive supervision. How can unlabeled video augment computational visual learning? I’ll describe our recent work exploring how a system can learn effective representations by watching unlabeled video. First we consider how the ego-motion signals accompanying a video provide a valuable cue during learning, allowing the system to internalize the link between “how I move” and “what I see”. Building on this link, we explore end-to-end learning for active recognition: an agent learns how its motions will affect its recognition, and moves accordingly. Next, we explore how the temporal coherence of video permits new forms of invariant feature learning. Incorporating these ideas into various recognition tasks, we demonstrate the power in learning from ongoing, unlabeled visual observations---even overtaking traditional heavily supervised approaches in some cases.