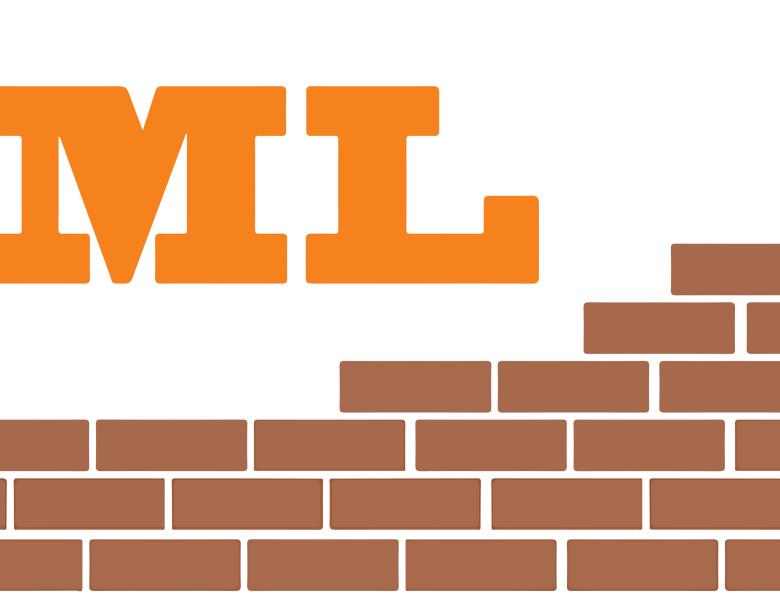
Abstract
Spectral methods provide remarkable theoretical and practical guarantees for the learning of latent variable models (LVM). In contrast to traditional likelihood based methods, spectral methods provide computationally tractable and space efficient algorithms which are accompanied by strong convergence guarantees. In this talk, we show how to deploy the power of spectral methods in Reinforcement Learning (RL) problems and derive efficient learning-planning algorithms in RL settings. In general RL applications, the assumption of full observability of all statistics fails, and we show how partially observable models (e.g. POMDPs, CMDPs) are able to provide suitable frameworks to model the environment by adding extra latent structures to generative models. In general, many challenges arise designing RL algorithms for partially observable models. We address those challenges and state how to utilize spectral methods to arrange efficient RL algorithms.