Image
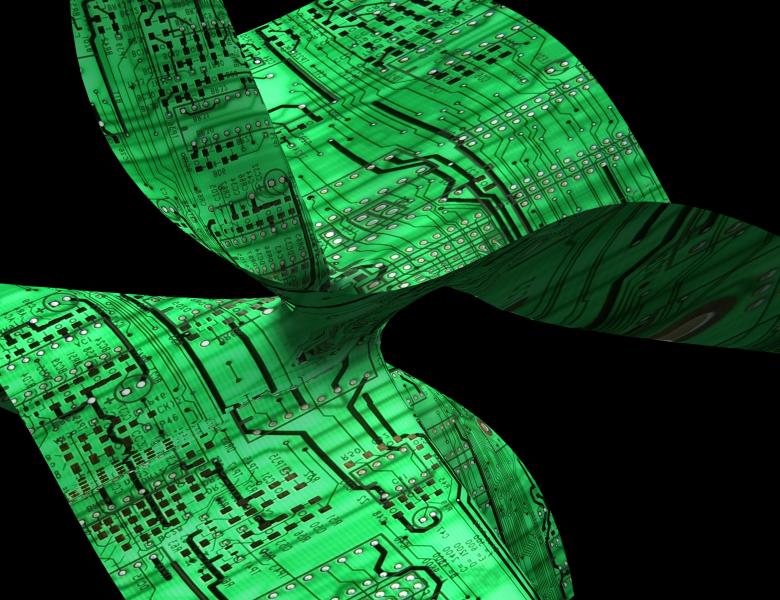
Numerical algebraic geometry is a growing area of applied algebra that focuses on describing solution sets of polynomial systems of equations. This area has already had an impact in kinematics, statistics, PDE's, and pure math.
In this talk, we focus on numerical algebraic geometry for maximum likelihood estimation in algebraic statistics. We motivate the problem with a specific example, present computational results consisting of ML degrees of determinental varieties, and a theoretical result of maximum likelihood duality.