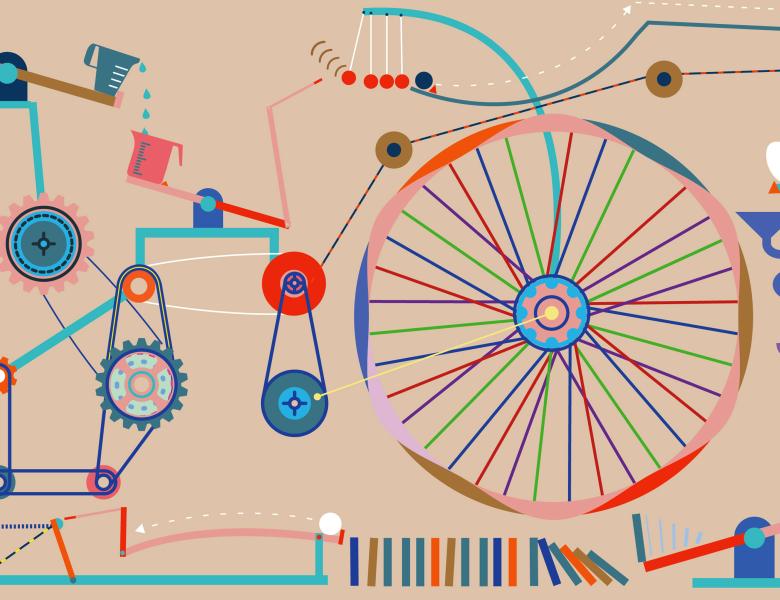
Abstract
Standard tools of causal inference such as do-calculus and identification algorithms require as input the articulation of assumptions in the form of causal diagrams. However, in many real-world applications, the knowledge necessary to specify a causal diagram over all variables is unavailable, particularly in complex, high-dimensional domains. We introduce a new graphical modeling tool called cluster DAGs (for short, C-DAGs) that allows for the partial specification of relationships among variables based on limited prior knowledge, alleviating the stringent requirement of specifying a full causal diagram. A C-DAG specifies relationships between clusters of variables, while the relationships between the variables within a cluster are left unspecified. C-DAGs can be seen as a graphical representation of an equivalence class of causal diagrams that share the relationships among the clusters. We develop the foundations and machinery for valid inferences over C-DAGs about the clusters of variables at each layer of Pearl's Causal Hierarchy - probabilistic, interventional, and counterfactual.