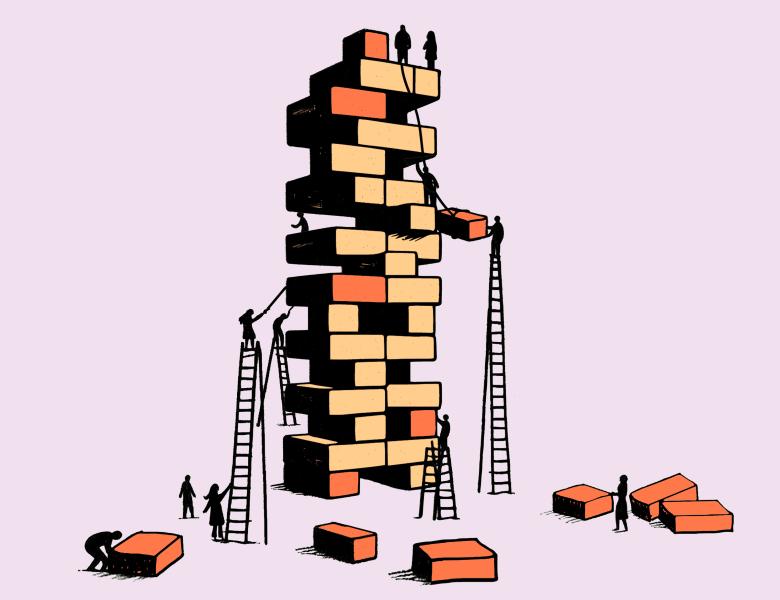
Abstract
Continuous optimization problems arise in virtually all disciplines of quantitative research. While convex optimization has been well studied in the past decades, nonconvex optimization generally remains intractable in theory and practice. In this talk, we introduce Quantum Hamiltonian Descent (QHD), a new quantum algorithm for continuous optimization. QHD is derived as the path integral of standard gradient descent (GD). It inherits the algorithmic simplicity of GD and meanwhile exhibits a drastically different behavior from GD due to the quantum interference of classical paths, especially for nonconvex optimization. Specifically, we prove that QHD can efficiently solve a family of nonconvex continuous optimization instances, each characterized by exponentially many local minima. The new mathematics of QHD, including a surprising connection between QHD and Wasserstein geometry, is yet to be understood.