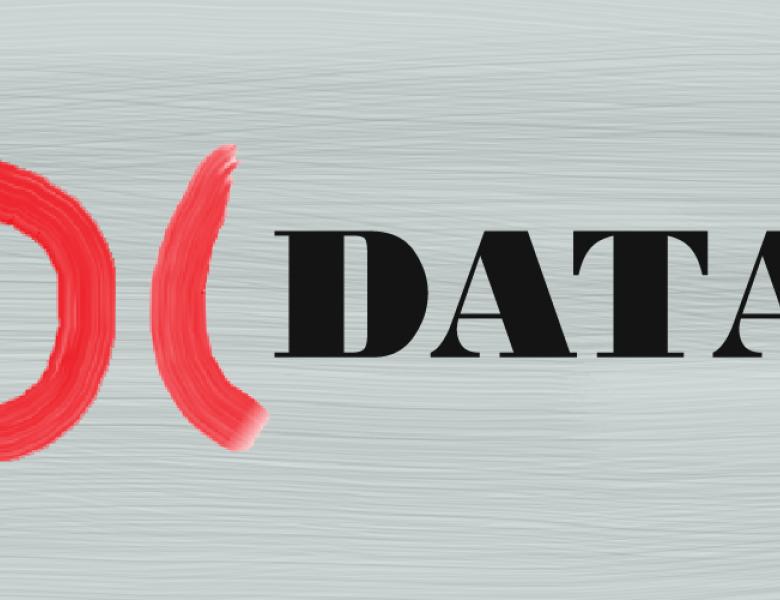
Abstract
Although network data offer several opportunities to improve prediction, the characteristics of real world datasets present a number of challenges to accurately incorporate relational information into machine learning algorithms. In this talk, I will discuss the effects of sampling, parameter tying, and model roll-out on the properties of the resulting statistical models--which occurs through a complex interaction between local model properties, global network structure, and the availability of observed attributes. By understanding the impact of these interactions on algorithm performance (e.g., learning, inference, and evaluation), we can develop more accurate and efficient analysis methods for large, partially-observable social network and social media datasets.