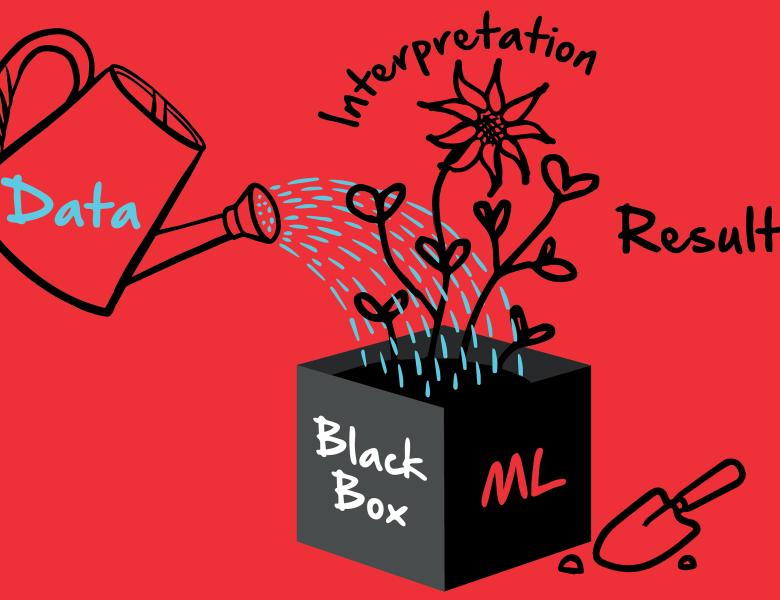
Abstract
Interpretability is a key component of many dimensions of building more trustworthy ML systems. In this talk, I will focus on a couple intersections between interpretability and algorithmic fairness. First, I will discuss some of the promises and challenges of causality for diagnosing sources of algorithmic bias. In particular, defining the nature and timing of interventions on immutable characteristics is highly important for appropriate causal inference but can create challenges in practice given data limitations. Second, I will discuss the strategy of collecting more diverse datasets for alleviating biases in computer vision models. Defining and measuring diversity of human appearance remains a significant challenge, especially given privacy concerns around sensitive attribute labels. To address this, I will present a method for learning interpretable dimensions of human diversity from unlabeled datasets.