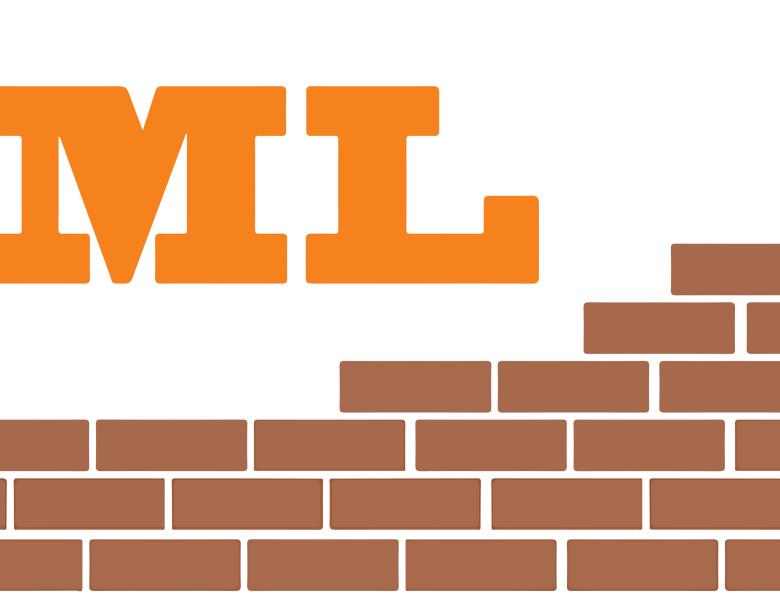
Abstract
We define an interactive setting for learning mixtures of submodular functions for the purpose of submodular summarization. Many previous methods to learn such mixtures require training sets that have been acquired offline. In our approach, the learner chooses the summaries and only minimal feedback is collected from (often human) evaluators, thereby reducing the arduous task of collecting evaluator-produced summaries. Our algorithmic framework involves, in each round, solving a difference of submodular (DS) optimization problem. We show that this framework can be slightly modified to handle variants more akin to regret minimization. We empirically demonstrate the efficacy on both synthetic data and for real-world applications, including speech data subset selection and image collection summarization. Improvements for these tasks are achieved by our method over a variety of baselines.
Joint work with Kai Wai, Wenruo Bai, and Rishabh Iyer.