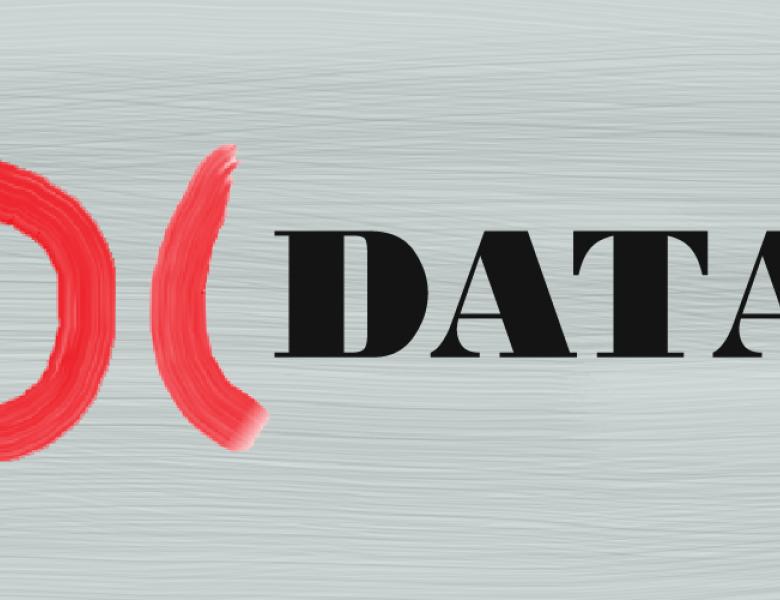
Abstract
The area of inference under shape constraints — that is, inference about a probability distribution under the constraint that its density function satisfies certain qualitative properties — is a classical topic in statistics. Shape restricted inference is well-motivated in its own right, and has seen a recent surge of research activity, in part due to the ubiquity of structured distributions in the natural sciences. The hope is that, under such structural constraints, the quality of the resulting estimators may dramatically improve, both in terms of sample size and in terms of computational efficiency.
In this talk, we will describe a framework that yields new, provably efficient estimators for several natural and well-studied classes of distributions. Our approach relies on a single, unified algorithm that provides a comprehensive picture of the sample and computational complexities for fundamental inference tasks, including density estimation and hypothesis testing.
The talk will be based in part on the following papers: http://www.iliasdiakonikolas.org/papers/piecewise-poly-learning.pdf, and http://www.iliasdiakonikolas.org/papers/identity-testing-structured.pdf.