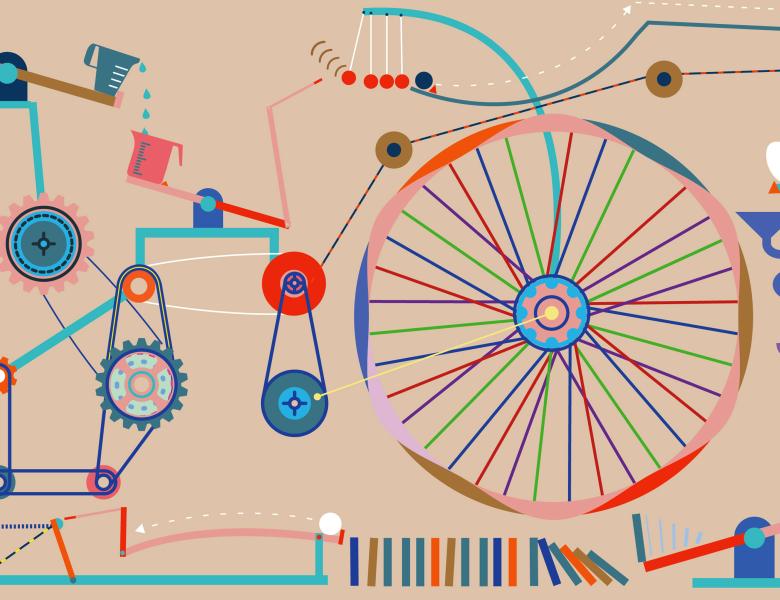
Abstract
We give an algorithm for source identification of a mixture of k product distributions on n bits. This problem (which we call k-MixProd) is a fundamental problem in machine learning with many applications. Our algorithm identifies the source parameters of an identifiable mixture, given, as input, approximate values of multilinear moments; the complexity is ~ exp(k log k) arithmetic operations and similar sample size. Our analysis gives a quantitative version of a qualitative characterization of identifiable sources, that is due to Tahmasebi, Motahari, and Maddah-Ali (2018). Key to our work are a method of ``synthetic bits'' and a condition number lower bound for Hadamard Extensions. Based on joint works with Chaitanya Swamy (Waterloo), Yuval Rabani (Hebrew U), Jian Li (Tsinghua), Spencer Gordon (Caltech) and Bijan Mazaheri (Caltech)