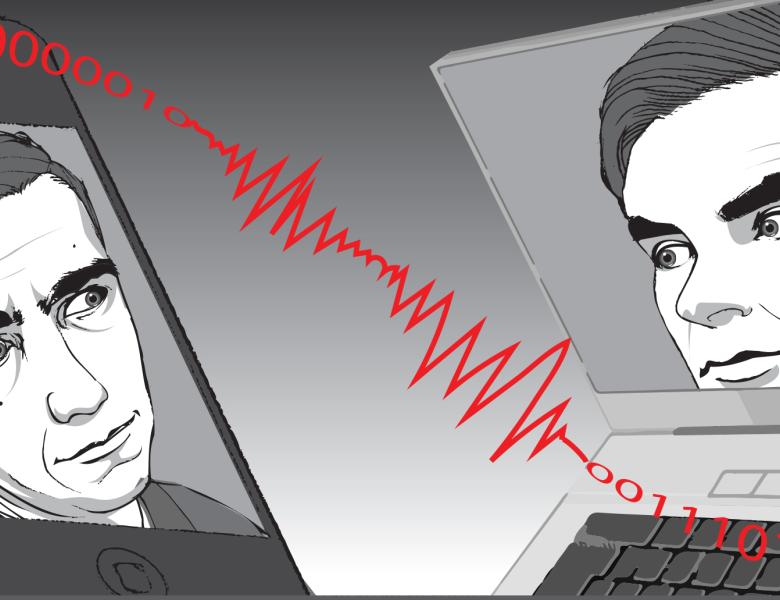
Abstract
Nonasymptotic estimates on the spectral norm of random matrices have proved to be indispensable in many problems in statistics, computer science, signal processing, and information theory. The noncommutative Khintchine inequality and its recent matrix concentration analogues are widely used in this context. Unfortunately, such simple bounds fail to be sharp in many applications. In this talk, I will discuss new bounds on the norm of random matrices with independent entries that are (provably) sharp in almost all cases of practical interest. Beside providing a substantial improvement on previously known results, the proof of these bounds is surprisingly illuminating and effectively explains when and why the norm of a random matrix is large. Joint work with Afonso Bandeira.