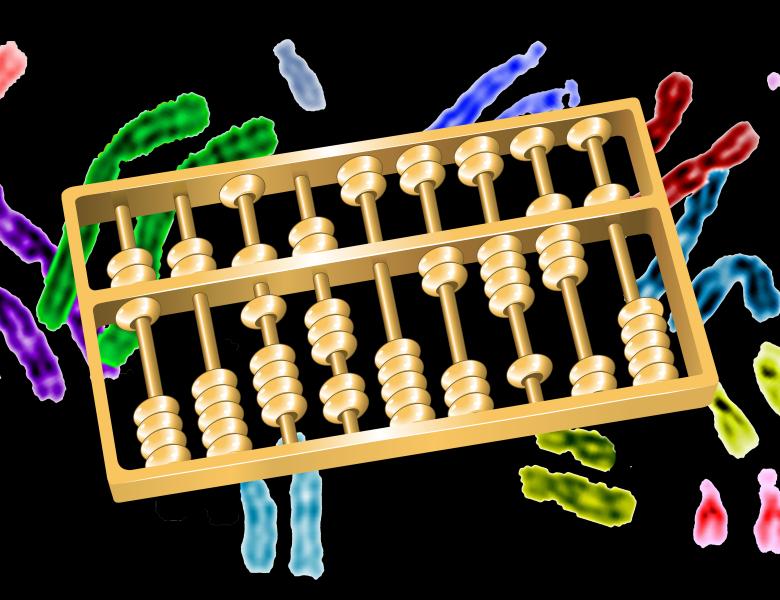
Abstract
Identification and prioritization molecular alterations that potentially act as drivers of cancer remain as a crucial challenge in cancer genomics and a bottleneck in the therapeutic development. The problem is particularly complicated by extensive mutational heterogeneity observed in the cancer (sub)types, yielding a long-tailed distribution of mutated genes across the patients, possibly implying the existence of many private drivers. In order to address this problem we have developed HIT’nDRIVE, a combinatorial algorithm that integrates genomic and transcriptomic (expression) data to identify patient-specific gene alterations that can collectively influence the dysregulated transcriptome of the patient. HIT’nDRIVE aims to solve the “random-walk facility location” (RWFL) problem on a gene/protein interaction network – thus differs from the standard facility location problem by its use of “hitting time”, the expected minimum number of hops in a random-walk originating from any sequence altered gene (i.e. a potential driver) to reach an expression altered gene, as the distance measure. Interestingly, hitting time when used as a distance measure, the distance between multiple facilities and a “target” is not the minimum distance. HIT’nDRIVE reduces RWFL (with multi-hitting time as the distance) to a weighted multi-set cover problem, which it solves as an integer linear program (ILP). Applying HIT’nDRIVE to 2200 (TCGA) tumors from four major cancer types has revealed many potentially druggable driver genes, several of which happen to be private. It is also possible to perform accurate phenotype prediction for these samples by only using HIT’nDRIVE implied driver genes and their “network modules of influence” (subnetworks involving each driver gene where the aggregate expression profile correlates well with the cancer phenotype) as features, providing additional evidence that these genes may be driving the cancer phenotype. Further analysis of these modules reveals patterns of mutual exclusivity among multiple driver genes modulating oncogenic or metabolic networks.