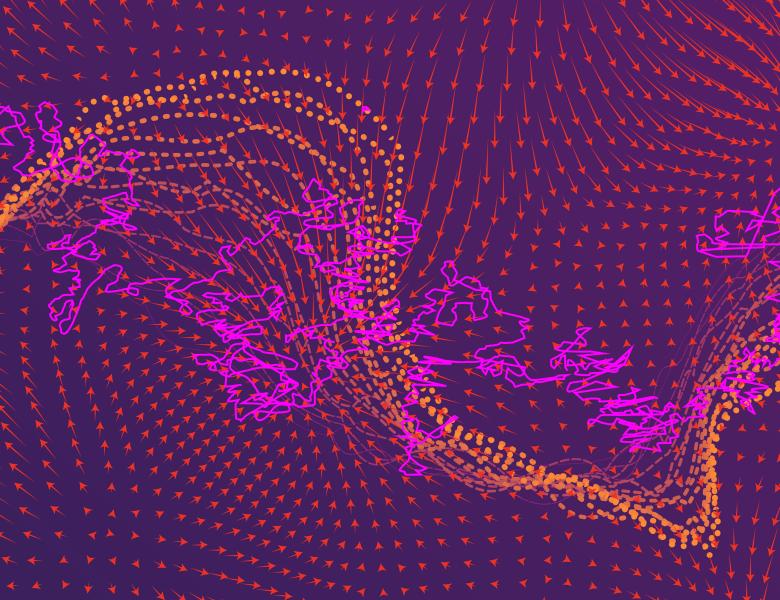
Abstract
Stochastic Gradient Descent (SGD) is widely used in modern data science. Existing analyses of SGD have predominantly focused on the fixed-dimensional setting. In order to perform high-dimensional statistical inference with such algorithms, it is important to study the dynamics of SGD under high-dimensional scalings. In this talk, I will discuss high-dimensional limit theorems and bounds for the online least-squares SGD iterates for solving over-parameterized linear regression. Specifically, focusing on the asymptotic setting (i.e., when both the dimensionality and iterations tend to infinity), I will first present the mean-field limit (in the form of infinite-dimensional ODEs) and fluctuations (in the form of infinite-dimensional SDEs) for the online least-squares SGD iterates. A direct consequence of the result is obtaining explicit forms and related fluctuations for the mean-squared estimation and prediction errors.