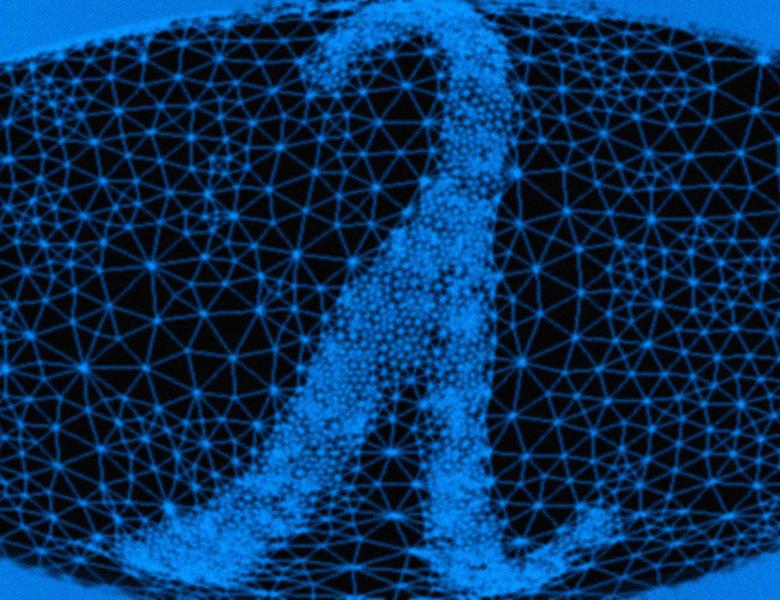
Abstract
Spectral clustering is a standard method for data analysis used in a broad range of applications. I will describe a new class of algorithms for multiway spectral clustering based on optimization of a certain class of functions after the spectral embedding. These algorithms can be interpreted geometrically as reconstructing a discrete weighted simplex. They have some resemblance to Independent Component Analysis and involve optimization of "contrast functions" over a sphere. However, in our case, theoretical guarantees can be provided for a much broader class of functions satisfying a "hidden" convexity condition. The algorithms are straightforward to implement, efficient and are not initialization-dependent.