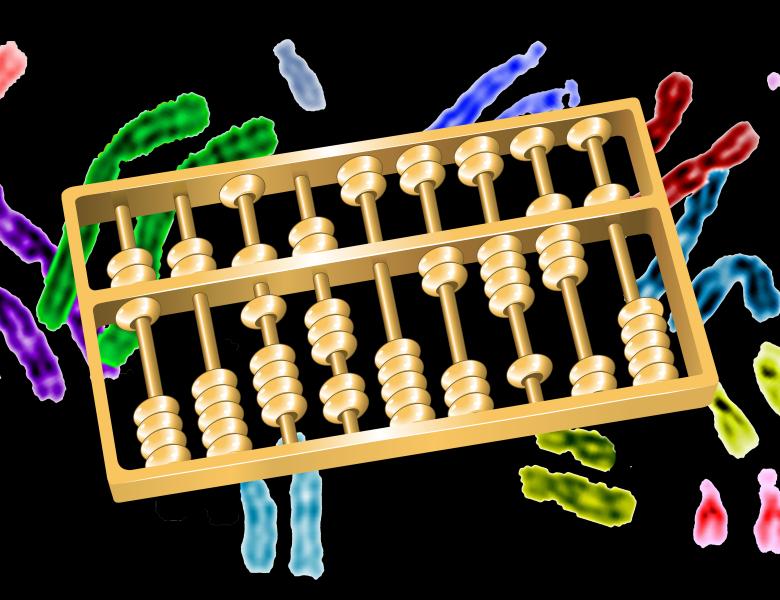
Abstract
SELEX-seq and HT-SELEX are sequencing-based methods for elucidating the intrinsic DNA binding specificity of transcription factor (TF) complexes at high resolution. While the amount of raw information that modern SELEX provides is unprecedented, the computational methods for building DNA recognition models (“motifs”) from these data are still far from mature. The standard is to tabulate of the relative enrichment of each oligomer of a given length, for which we have developed efficient software. Unfortunately, having to use oligomer tables as an intermediate step for feature-based analysis has two key disadvantages: (i) limited range over which readout can be analyzed, as counts decrease exponentially with footprint size; and (ii) requirement for prior ad hoc sequence-based alignment of different oligomers. We present a new and versatile framework for motif discovery from SELEX data that overcomes these limitations. It uses a hierarchical maximum likelihood approach to fit a feature-based biophysically motivated protein-DNA recognition model directly to the raw SELEX data. This allows us to consider base and shape readout in more detail and over a larger footprint than was possible before, which we illustrate using data for the steroid hormone receptors AR and GR. We can now for the first time analyze shape readout for TFs with low binding specificity, which we demonstrate using Hox monomer data.