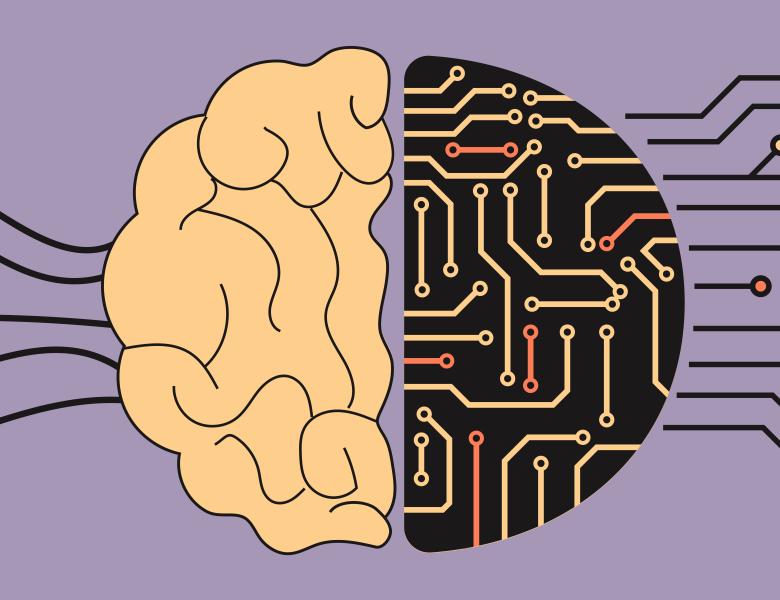
Abstract
The quality of generative models depends on the quality of the data on which they are trained. Access to high-quality data is scarce and expensive, while noisy samples are generally more accessible. State-of-the-art generative models are often trained on curated datasets that emerge from highly filtered data pools from the Web and other sources. In this talk, we will show that there is immense value in the lower quality data that are often discarded. We will present an algorithmic framework to train generative models using a combination of a small set of expensive, high-quality samples and a large set of cheap, noisy points. Our framework is instantiated for diffusion generative models, specifically through our Ambient Diffusion method. We will show how Ambient Diffusion enables training on noisy images and that it achieves state-of-the-art performance in de novo protein design. Time permitting, we will also present preliminary extensions to autoregressive language modeling and discuss broader implications for memorization, dataset design, and model performance.