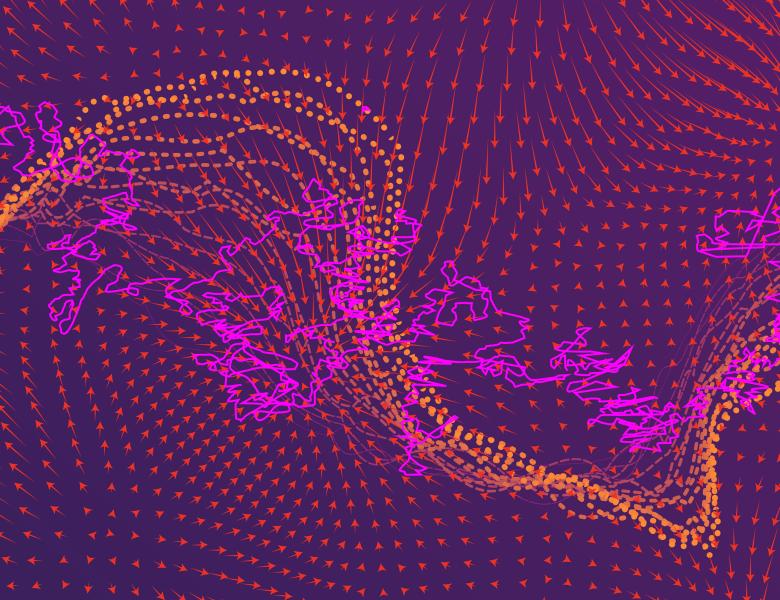
Abstract
In this talk I will propose new tools for the exploratory data analysis of data objects taking values in a general separable metric space. I will introduce depth profiles, where the depth profile of a point ω in the metric space refers to the distribution of the distances between ω and the data objects. I will describe how depth profiles can be harnessed to define transport ranks, which capture the centrality of each element in the metric space with respect to the data cloud. Finally, I will discuss the properties of transport ranks and show how they can be an effective device for detecting and visualizing patterns in samples of object data.