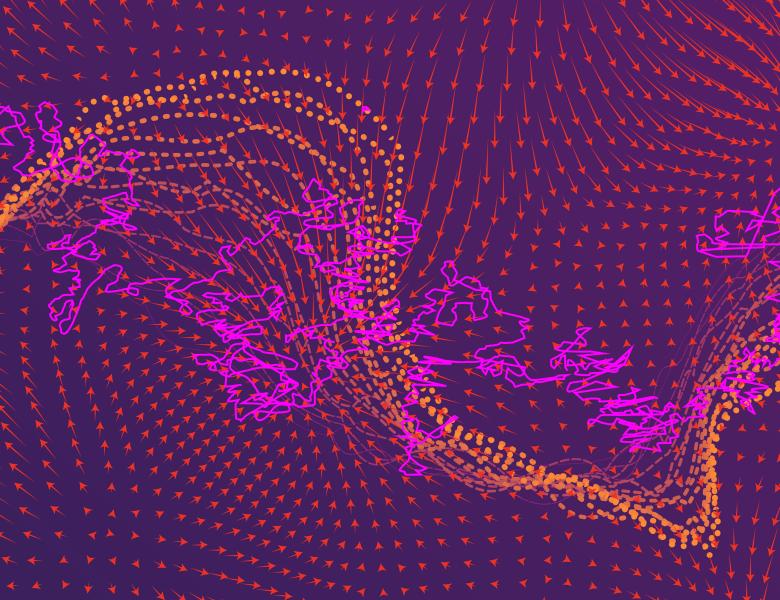
Abstract
In this talk I will show that Adversarial Training—a robust optimization method designed for the training of adversarialy robust classifiers— is equivalent to a variational regularization problem involving a nonlocal and data-dependent perimeter term. Using this structure one can show that adversarial training of binary classifiers admits a convex relaxation which is reminiscent of the Chan–Esedoglu model from image denoising. Furthermore, this allows to prove existence of solutions and study finer properties and regularity. Finally, I will discuss Gamma-convergence of the nonlocal perimeter, as the strength of the adversary tends to zero, to an isotropic local perimeter. This talk is based on joint work with Nicolás García Trillos and Ryan Murray, which started at Simons GMOS in 2021, and with Kerrek Stinson.