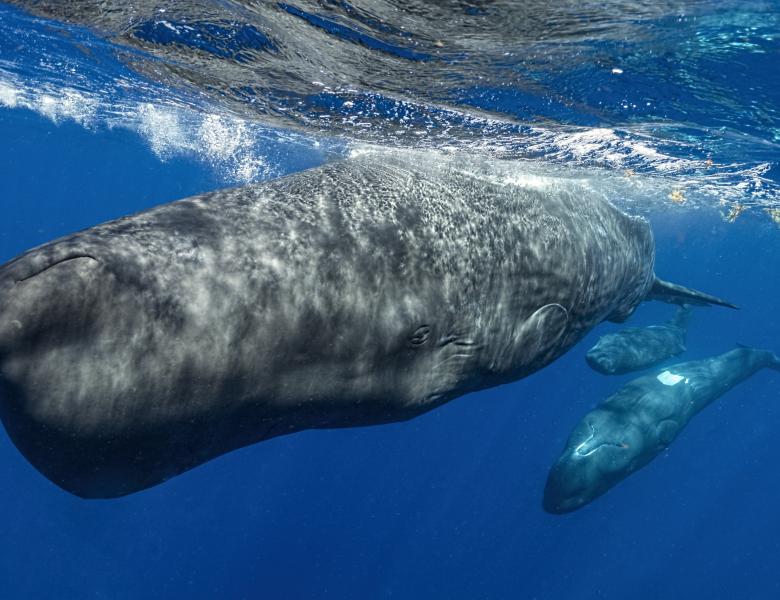
Abstract
Interpretability is the new frontier in AI research. Understanding how generative models learn and how they resemble or differ from humans can not only provide insights for the study of human language and cognition, but can also facilitate discovery of novel patterns in diverse fields. In this talk, I outline a more realistic model of human language acquisition and introduce an AI interpretability technique that allows us to establish a causal relationship between individual neurons and linguistically meaningful properties. Using the proposed technique, we can compare and evaluate artificial and biological neural processing of language. Additionally, I show that AI interpretability techniques can facilitate scientific discovery by uncovering previously unrecognized patterns in complex data types. I will argue that sperm whales have analogues to human vowels. This discovery was predicted, but not fully described, by the proposed AI interpretability technique. I will also show that the co-called coda vowels feature several behavioral parallels with human vowels, such as coarticulation and prominence in sub-coda structure.