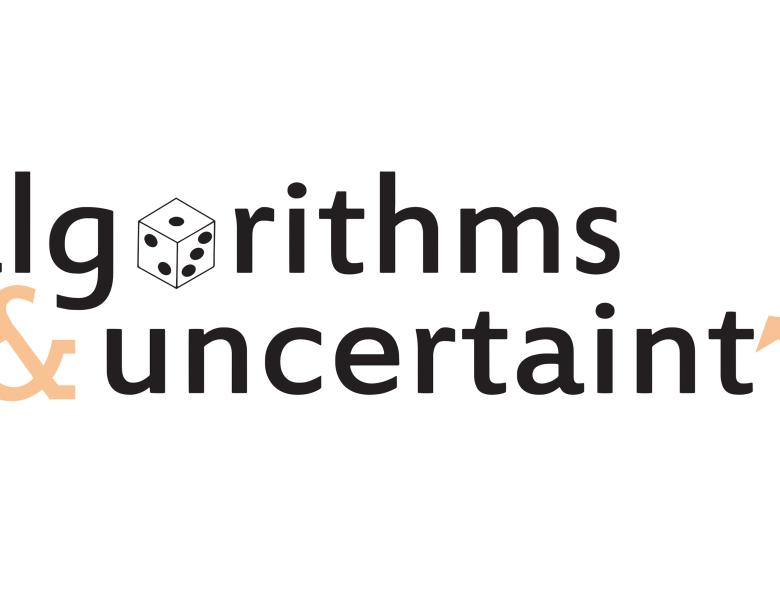
Abstract
We investigate dropout for a single neuron (inputs are dropped independently at random with probability half). When the loss is linear, then we can prove very good properties for dropout perturbation: optimal regret in the worst case without having to tune any parameter, AND optimal regret in the iid case when there is a gap between the best and second best feature.
We give high level intuitions of the new proof techniques and discuss a number of competitor algorithms some of which require tuning.
Joint work with Tim Van Erven and Wojciech Kotlowski.