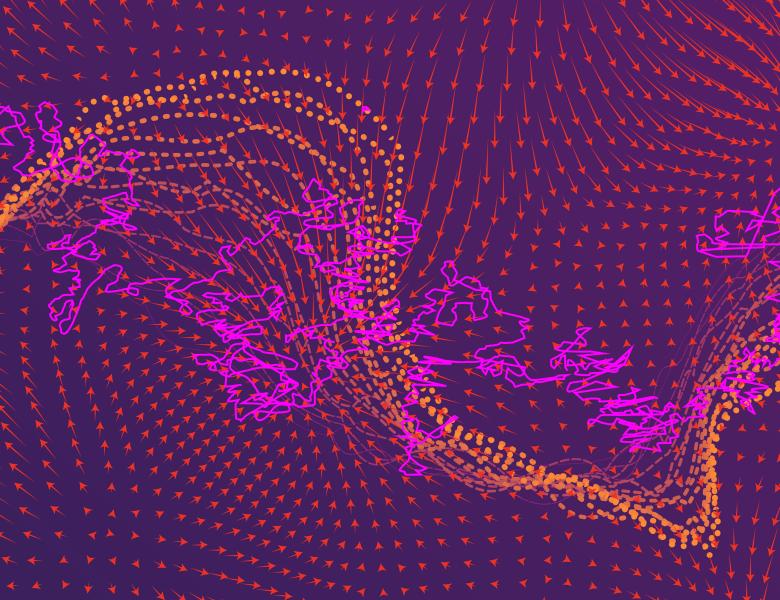
Abstract
We study the effect of gradient-based optimization on feature learning in two-layer neural networks. We consider two settings: 1- In the proportional asymptotic limit, we show that the first gradient update improves upon the initial random features model in terms of prediction risk. 2- In the non-asymptotic setting, we show that a network trained via SGD exhibits low-dimensional representations, with applications in learning a single-index model with explicit rates.