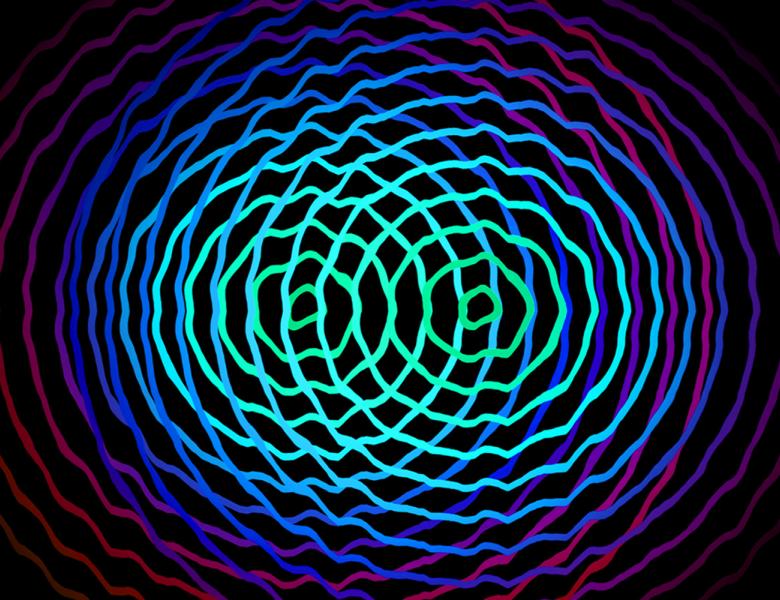
Abstract
We study the power of quantum memory for learning properties of experimental systems, in particular quantum systems and their dynamics. By synthesizing and generalizing recent approaches to quantum learning algorithms and quantum algorithmic measurements, we provide a new framework for proving exponential complexity separations between interactive protocols with and without quantum memories. We will establish (i) exponential separations between algorithms with and without quantum memory for purity testing, distinguishing scrambling and depolarizing evolutions, as well as uncovering symmetry in physical dynamics; (ii) exponential tradeoffs between quantum memory and sample complexity for estimating Pauli observables; and (iii) tight bounds (up to logarithmic factors) for shadow tomography without a quantum memory. Some of our complexity advantages could be realized on NISQ devices with tens of qubits, providing a concrete path towards demonstrating a real-world advantage for learning algorithms with a quantum memory.