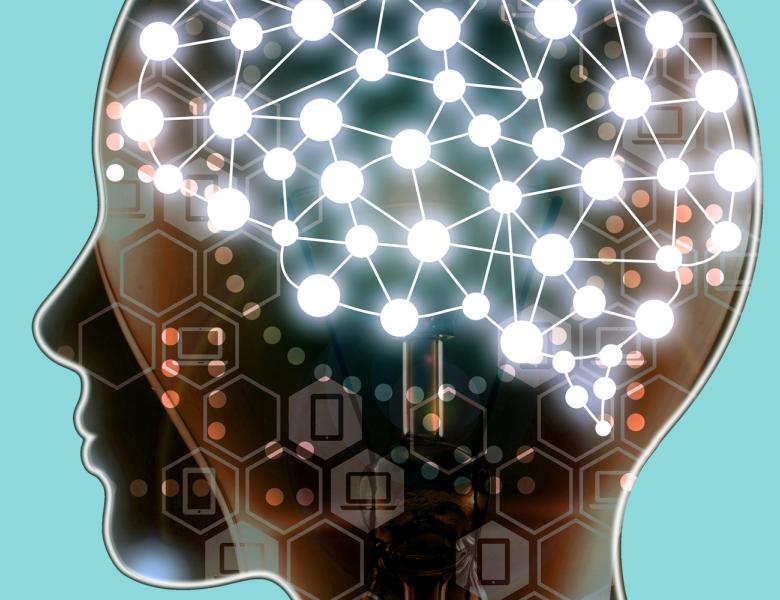
Abstract
We consider a class of supervised learning problems whereby we are given n data points (y_i,x_i), with x_i a d-dimensional dfeature factor, y_i a response, and the model is parametrized by a vector of dimension kd. We consider the high-dimensional asymptotics in which n,d diverge, with n/d and k of order one. As a special case, this class of models includes neural networks with k hidden neurons.
I will present two sets of results:
1. Universality of certain properties of empirical risk minimizers with respect to the distribution of the feature vectors x_i.
2. A sharp asymptotic characterization of gradient flow in terms of a one-dimensional stochastic process.
[Based on joint work with Michael Celentano, Chen Cheng, Basil Saeed]