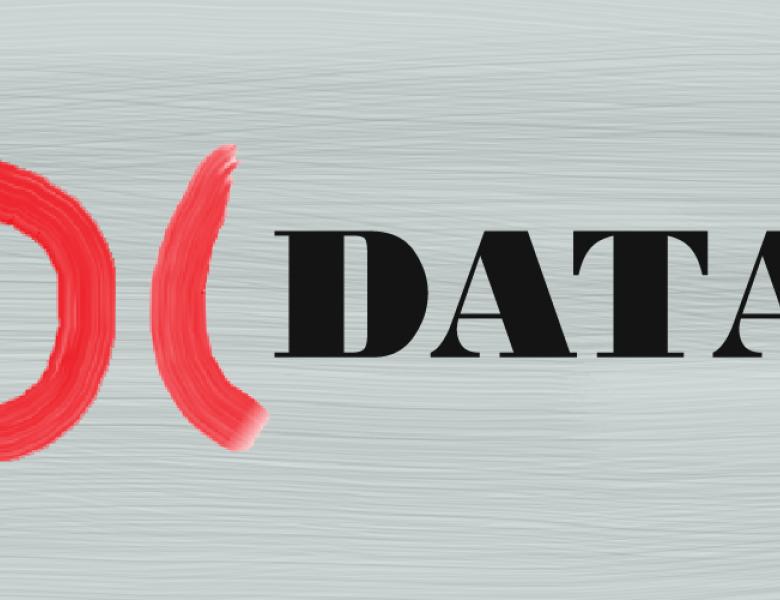
Abstract
Extracting knowledge and providing insights into the complex mechanisms underlying noisy high-dimensional data sets is of utmost importance in many scientific domains. Networks are an example of simple, yet powerful tools for capturing relationships among entities over time. I will present a line of work that deals with the estimation of high-dimensional dynamic networks from limited amounts of data. In particular, I will present a general framework of flexible semiparametric models, capable of capturing the dynamics of network changes, and efficient estimation procedures tailored to different assumptions on the underlying dynamics. Illustrative applications of exploring complex real worlds systems will be presented as well.